Most fraud prevention and detection tools deliver results via fraud scores, but what do they measure, and how exactly do they work? In this article, we’ll break down the basics of fraud scoring and explore how you can leverage them to boost business efficiency.
What Is a Fraud Score?
A fraud score is a number that answers the question, “How likely is this person to be a fraudster?” Fraud scoring assigns a value to how risky a user action is. The fraud scores are calculated using rules which add or subtract points based on the known data points about a user.
For instance, the user action may be a signup, login or card payment. Known data points include the user’s IP address, email address, or their device configuration.
In fact, there are dozens of different data points within each of these. An email address can appear on known blacklists, for example. Or an IP address, for instance, can be tied to known Tor nodes or locales. In fact, note that an IP fraud score is its own specific kind of fraud score.
How Does Fraud Scoring Work?
Fraud scoring is the process of assessing how risky a user action is, based on available data and predefined rules. Much like a credit bureau evaluates financial risk, a fraud prevention software calculates a risk score by analyzing signals tied to user behavior and identity. Based on the result, actions can be automatically approved, rejected or sent for manual review.
This is how it looks in practice:
- A user attempts an action, such as registering, logging in or making a payment.
- The system gathers data from submitted fields (e.g., email, phone number) and technical signals (e.g,. device type, IP address).
- A data enrichment process expands this dataset using digital footprint analysis, device intelligence and external sources to reveal patterns, digital presence and risk markers.
- All data is passed through the scoring engine, where rules assign weighted values (positive or negative) to each signal.
- The final fraud score is calculated, with transparent logic available if a whitebox machine learning is in use.
- Based on configured thresholds, the system triggers one of three actions: Approve, Decline or Manual Review.
Here are some key features to look for in a granular scoring system:
- Every datapoint used in scoring is fully visible and can be exported for analysis.
- Risk rules are completely customizable: you choose which are active, how much they influence the score and under what conditions they apply.
- You can build your own rules, import industry-standard rules and organize them into categories for clarity.
- Machine learning modules analyze user behavior over time and suggest new rules to improve accuracy, with options for manual approval or auto-deployment.
Fight fraud with fully transparent risk scoring and powerful rulesets using machine learning and human insight.
Ask an Expert
Fraud Score Calculation Example
Consider a scenario where a user attempts to complete a payment on your platform. As the transaction is processed, the fraud scoring engine evaluates a series of risk signals and applies relevant rules, each contributing to the final score.
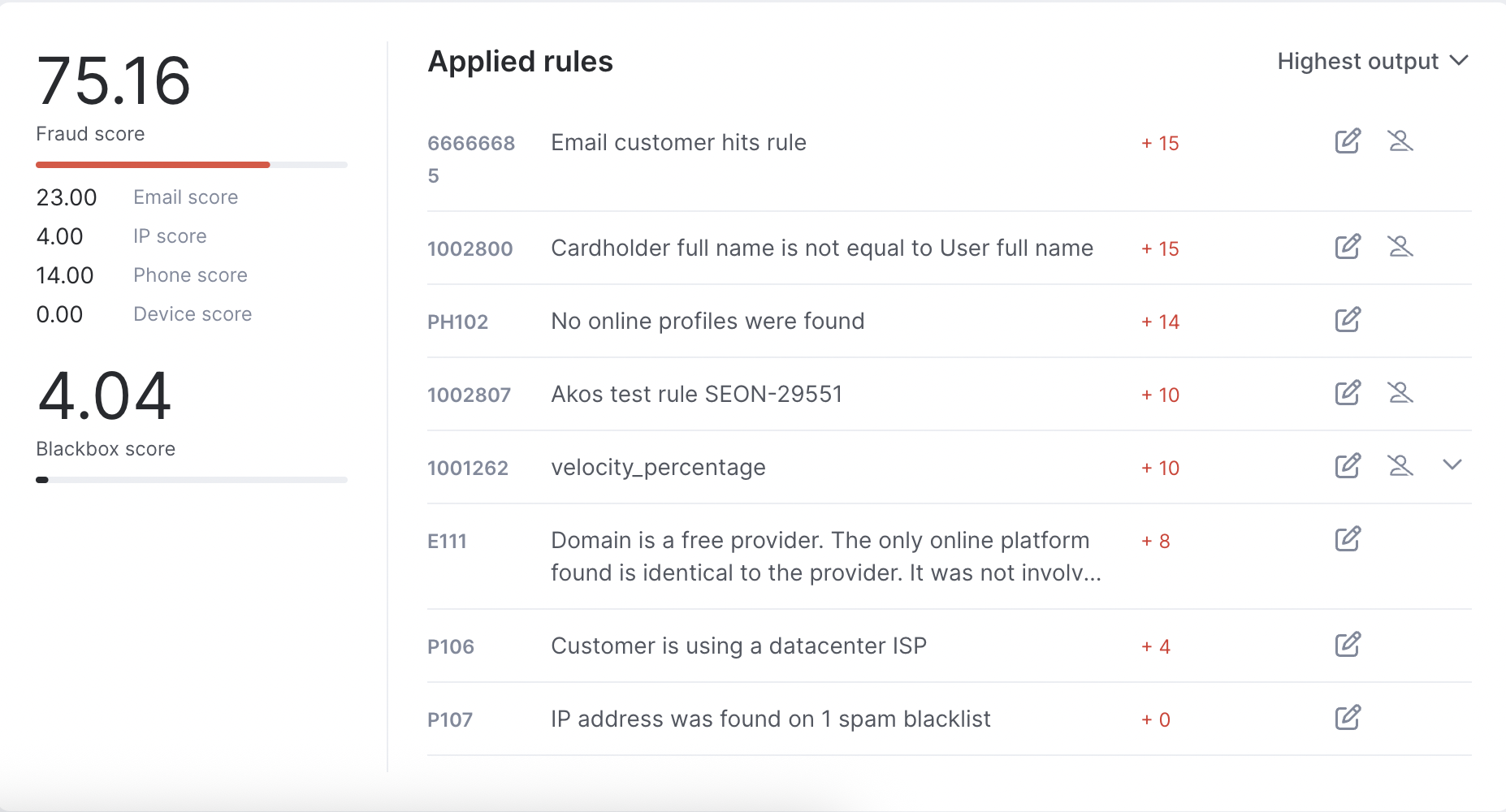
In this case, several risk signals are detected: the user triggers email- and identity-related rules, shows a limited digital footprint and exhibits unusual behavioral patterns. Additional risk is flagged based on the use of a free email domain and a datacenter IP, both common among suspicious or automated activity.
Altogether, these factors result in a fraud score of 76 out of 100, signaling a high-risk transaction. Depending on the organization’s scoring thresholds, this could trigger an automatic rejection or flag the transaction for manual review.
What makes this process powerful is its configurability. You might choose to automatically block all transactions scoring above a certain threshold or only escalate borderline cases for human review. This flexibility allows the scoring system to align with your business logic and evolving fraud landscape, making fraud prevention not only effective but also adaptive to your specific needs.
Advantages of Fraud Scoring
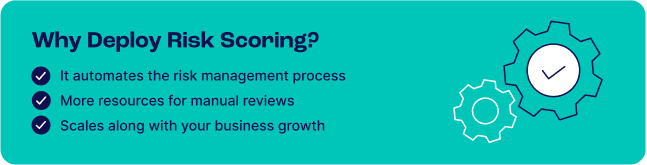
To understand the benefits of fraud scores, let’s imagine you are a small online store focusing on reducing transaction fraud (when users pay with stolen credit card details).
Your goal is to reduce chargebacks, identify legitimate users, weed out fraudsters, and facilitate good payments. So, what can fraud scores do for you?
- They allow automation: Instead of manually reviewing every purchase, you can let the system assign a value to each action, and approve or deny it based on the results. Of course, you can also review actions where the results are indecisive for certain transactions.
- Scaling: Fraud scores will let your store process many more transactions, more quickly. This helps you focus on growing your business with complete peace of mind, while risk management is taken care of in the background.
- Dynamic authentication: Even if your risk numbers point to the need for manual review, you can still add another layer of safety with triggers. Let’s say someone signs up to your platform, but their transactions data signals they might be a risky user. Your risk prevention system could trigger additional authentication such as 2FA, which can confirm their identity, and deter potential fraudsters.
- Reduced friction and customer churn: When you automate reviews with risk scores, you create a smoother customer journey. For instance, Amazon doesn’t ask for a credit card CVV to speed up the payment process. You can reduce the number of steps between your user and their payment, as long as only risky behavior is reviewed.
- Better flexibility: Balancing the numbers yourself lets you decide how you want to mitigate risk. This could be based on seasonality, or for specific items, such as high-value goods or low-value digital downloads. Just keep in mind that not all fraud prevention tools let you adjust the thresholds yourself.
Disadvantages of Fraud Scoring
The biggest disadvantage of fraud scoring is that no two companies use the same standards, so switching providers often means relearning how to assess risk. For example, a score of 0 could mean an excellent user for one provider but a risky one for another. At SEON, we offer preset thresholds to help you get started — but you can easily customize them at any time.
0 to 10: | The action is safe and can be approved automatically. |
10 to 20: | The action could be risky, and should probably be reviewed manually. The user journey is momentarily paused, and you can create an alert via email, for instance, to manually process the action. Another option is to trigger a second set of verifications automatically at this stage. This dynamic friction strategy will help you reduce false positives. |
20 or more: | The action is risky and will be declined. You can blacklist the user’s data points forever if you want. |
All of these can be adjusted manually. But before tweaking them, it’s important to first get a good understanding of which rules give us a fraud score.
How to Get Started with Fraud Scoring
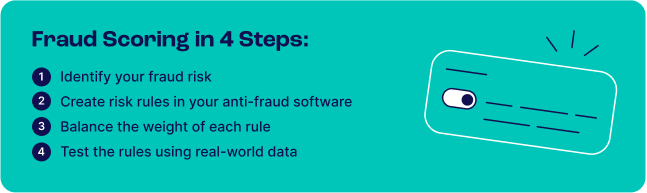
Fraud scoring can vary significantly across tools, so understanding its foundational elements is essential before implementing or selecting a solution. Here are some key areas to focus on:
1. Understand Where the Fraud Rules Come From
Fraud scores are calculated based on rules that may come from multiple sources:
- Predefined by the provider and tailored to common industry risks
- Manually created to reflect business-specific scenarios
- Suggested by machine learning based on historical data patterns
However, fraud prevention isn’t one-size-fits-all. A rule that’s effective for a crypto platform might be irrelevant — or even disruptive — in an iGaming or eCommerce context. That’s why rules should always be tested against real historical data within your business environment.
In the case of machine learning–generated rules, transparency matters: being able to interpret why a rule is suggested (and what it does) is critical for trust and operational control. This is where whitebox systems provide a clear advantage.
2. Consider Whitebox vs Blackbox Approaches
Machine learning algorithms applied in fraud scoring engines typically fall into two categories:
- Whitebox models offer full transparency into how risk scores are calculated, letting you see and control the logic behind each rule and threshold.
- Blackbox models use advanced machine learning to predict risk but don’t expose the underlying decision logic, making them harder to interpret or audit.
Whitebox systems are ideal for teams that need explainability, auditability and flexibility, allowing you to adjust rule weights, test logic and define custom thresholds based on your risk appetite.
However, the most effective approach is to combine both models. Whitebox scoring ensures control and clarity, while blackbox models surface hidden threats and emerging fraud trends. Together, they offer a balanced, high-precision framework for adaptive fraud prevention.
3. Test the Rules for Accuracy
Fraud scoring accuracy is only as strong as the data it’s built on. That’s why it’s essential to use a system that not only captures a wide range of datapoints but also enriches them with external context.
Effective fraud scoring solutions should:
- Validate and assess the quality of user-submitted data
- Cross-reference inputs (like email, IP or device) with external sources to uncover risk signals
- Minimize the amount of information required from users without sacrificing detection quality — streamlining onboarding and reducing friction
By ensuring that both the data and the rules are tested, contextualized and flexible, you lay the groundwork for a scoring model that adapts to your needs and catches fraud with greater precision.
How Does Fraud Scoring Work at SEON?
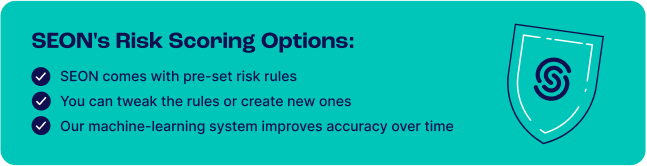
SEON’s fraud scoring is transparent and fully customizable. It combines human-defined rules with whitebox and blackbox machine learning models. Designed to support a broad range of industries and risk strategies, it lets users define their own rules while offering intelligent automation for those who prefer a hands-off approach.
When going into the Scoring Engine from the Admin panel, users can view, edit or add rules, while also accessing machine learning-driven insights based on historical data to optimize fraud detection.
1. Default Rules
Default rules represent SEON’s baseline recommendations, created by fraud analysts based on common fraud patterns observed across industries. To simplify navigation, these best-practice rules are grouped by category, such as email, IP and phone.
For instance, a rule might add +10 points to the score if a disposable phone number is used. In contrast, detecting a remote access protocol may only add +1 point. That’s because, in isolation, it’s not a strong fraud signal — but if multiple risk factors are present, the total score may exceed the review or block threshold.
The first column in the rule list includes a toggle switch, allowing users to easily enable or disable individual rules.
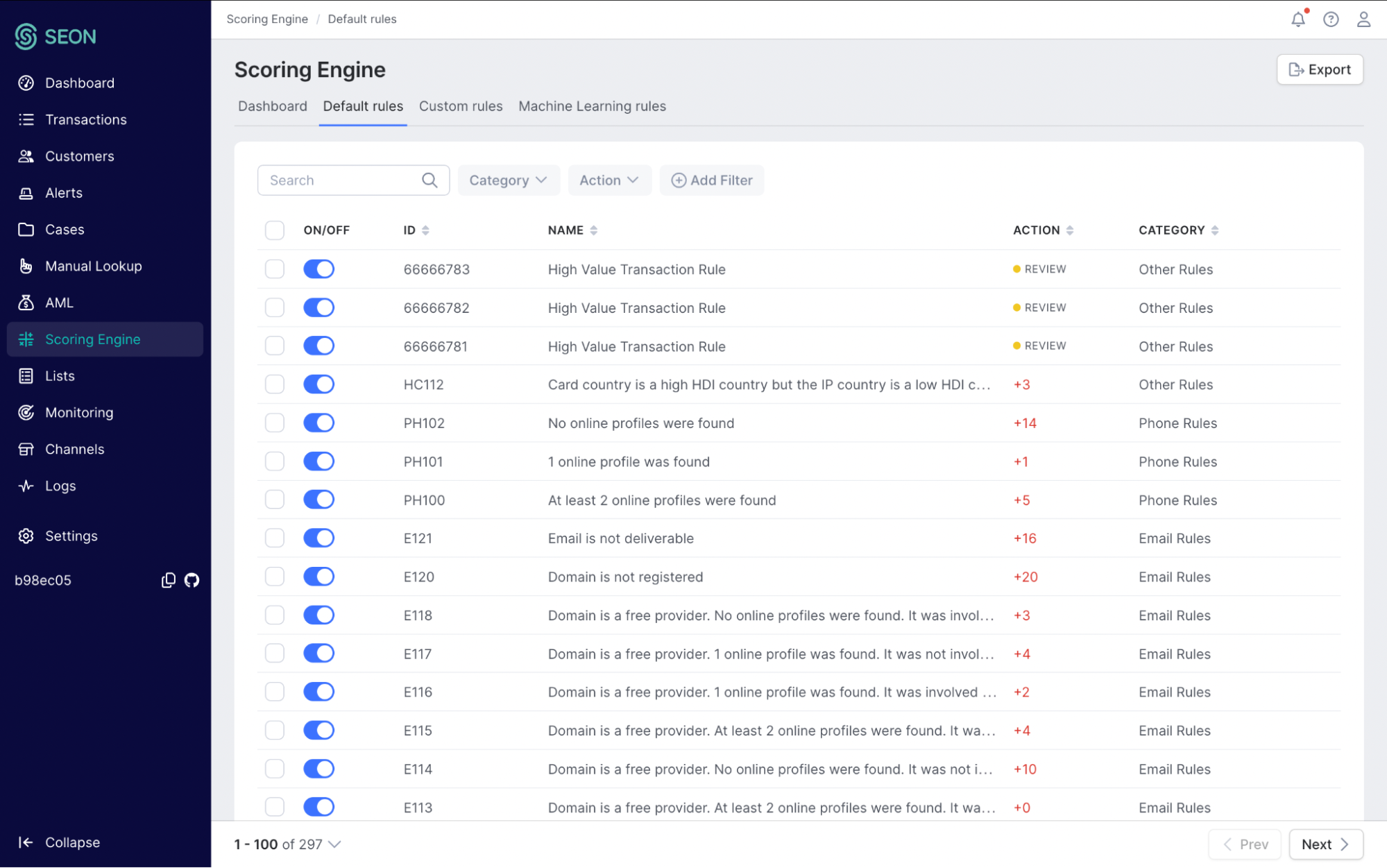
2. Custom Rules
Custom rules give SEON users complete control over fraud scoring, allowing them to define logic tailored to their risk appetite, industry and workflows. Each rule can trigger specific actions — adjusting the score, changing the transaction state (Approve, Review, Decline) or managing user lists (Blacklist/Whitelist) — with full control down to decimal-point precision.
Rules are built using one or more of the following parameter types:
- Data match: Checks for exact value matches (e.g., flagging devices at 0% battery).
- Compare: Uses standard operators like greater than, exists or equals (e.g., subtracting points for users with multiple social profiles).
- Velocity: Analyzes behavior over time (e.g., blocking IPs used by 15+ users within 30 minutes).
Users can combine multiple conditions, group rules into custom categories and use templates to speed up setup. All rules are searchable and testable against historical data via a built-in preview feature or the sandbox environment, making it easy to finetune risk strategies with confidence.
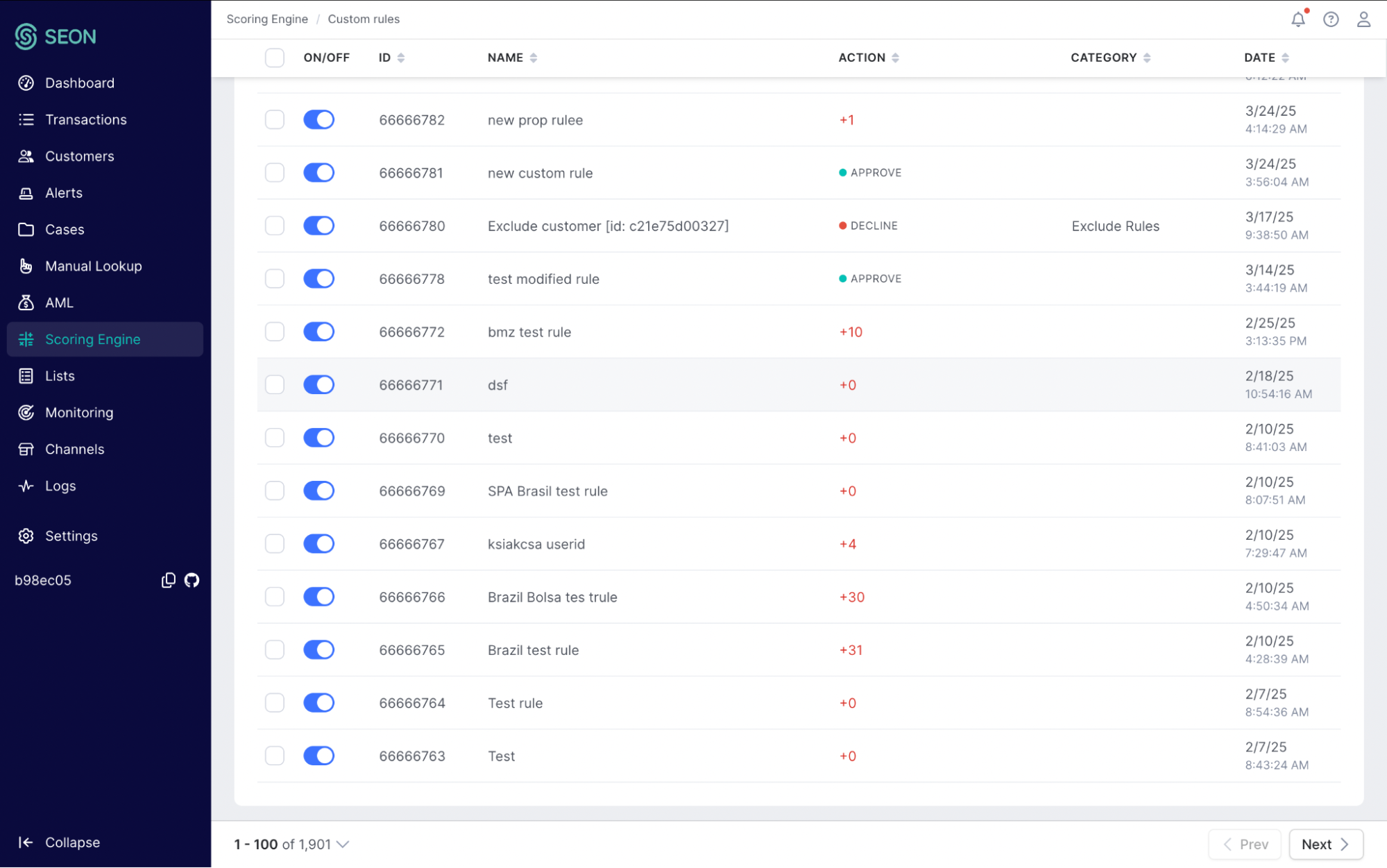
3. Machine Learning Rules
SEON’s whitebox machine learning module generates rule suggestions based on patterns it detects from historical data and user-labeled decisions. These rules are fully transparent, with each suggestion showing its logic and an associated confidence score, so you understand why it was recommended and how reliable it is.
Over time, the model learns from your team’s Approve/Review/Decline actions and uncovers behavioral trends across both legitimate users and fraudsters. Suggested rules can be reviewed, edited or enabled directly in the Scoring Engine.
For a more automated setup, you can choose to auto-activate machine learning-generated rules above a set confidence threshold (or all of them), streamlining fraud prevention while maintaining visibility and control.
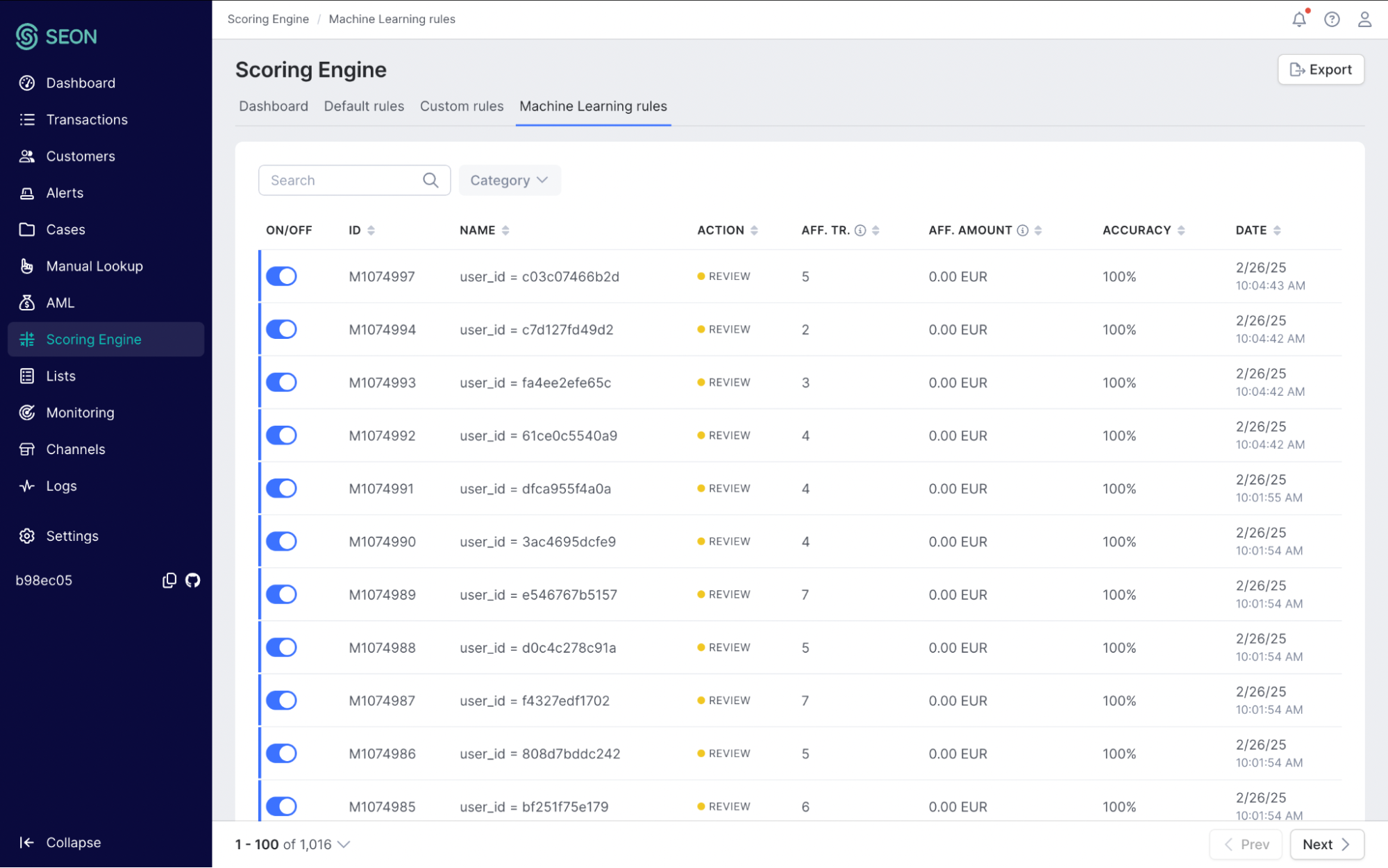
4. Industry Presets
In addition to SEON’s transparent (whitebox) fraud scoring, the platform also offers a powerful blackbox machine learning module that independently assesses the probability of fraud for each transaction. This system operates in the background, identifying subtle patterns and evolving fraud tactics that may not yet be covered by rule-based scoring.
Once enabled, Blackbox scoring starts assigning a separate risk score, from 0 to 100, to each transaction. This score is based on SEON’s continuously trained machine learning model, which draws on global fraud data and customer-specific trends. While the logic behind the score is not exposed, it offers valuable predictive power from day one.
Blackbox scores can also be incorporated into custom rules, for example, automatically flagging or reviewing transactions with a blackbox score above 30, further enhancing customer risk assessment and decision automation.
Fraud Scoring Example Workflow
SEON’s fraud scoring engine draws on hundreds of data points via digital footprint assessment and device intelligence to deliver precise, real-time risk evaluations. Here’s how that process works in practice:
- User signs up: A user registers on your platform, providing only a name and email address.
- Automated enrichment begins: In the background, SEON gathers data via digital footprint and device analysis, uncovering details such as IP address, device type, browser version and linked social media accounts based on the email or phone number.
- Cross-referencing external data: This raw data is enriched using external sources to reveal insights like the email’s domain quality, presence on blacklists or history of use across platforms.
- Scoring engine activation: The enriched dataset is passed through the rule-based and machine learning scoring engines.
- Risk score output: A fraud score is returned, helping determine whether the user should be approved, reviewed or blocked.
Skipping enrichment steps would limit visibility into the user’s digital footprint, weakening the effectiveness of your customer risk assessment and potentially allowing fraudulent behavior to slip through.
Key Takeaways
A robust fraud scoring strategy relies on the combination of two critical elements: transparency and adaptability. This is best achieved by integrating both whitebox and blackbox machine learning models and custom risk rules.
Whitebox models provide clarity and control, allowing fraud teams to define, adjust and audit scoring rules based on clear logic and explainable outcomes. Blackbox models, on the other hand, deliver dynamic, data-driven insights. By analyzing historical patterns and user-labeled outcomes, these systems uncover correlations and emerging fraud trends that static rule sets might miss. Even though the logic behind their scores isn’t visible, they provide valuable signals that enhance detection accuracy from the outset.
Together, these approaches offer a complementary framework: one that empowers organizations to understand and shape their fraud prevention strategy while also staying ahead of evolving threats. The ability to customize risk rules, incorporate machine learning and adjust thresholds to fit business needs is what distinguishes effective, modern fraud prevention systems.
SEON gives you full control over fraud scoring, combining transparent rule logic with powerful machine learning to stop threats before they impact your business.
Speak with an Expert
Frequently Asked Questions
Fraud scores are calculated by feeding user data through risk rules. The total score should fall within a range that lets you know whether you should accept, decline, or review the action.
It depends. Generally, longer rules weigh down the system more than more rules. In other words, longer rules that involve more parameters will have a greater impact on performance than shorter rules. Sometimes, an easy solution to this is to break them up into shorter, simpler rules. Made up of fraud managers and analysts exclusively, SEON’s Customer Success team can help you identify any such rules and optimize them to speed up your operations.
Fraud scoring can give out results with extremely high accuracy. However, the quality of the results depends on the kind of rules in place, how up to date they are, and even what kind of industry you are in.
Further reading:
Learn more about:
Browser Fingerprinting | Device Fingerprinting | Digital Footprinting | Fraud Detection with Machine Learning & AI