Credit card fraud is on the rise and, according to the Nilson Report, it’s projected to reach a staggering $38.5bn by 2027. So how do you detect credit card fraud? And why is it so common? Find all the answers below.
What Is Credit Card Fraud Detection?
Credit card fraud detection is a set of methods and techniques designed to block fraudulent purchases, both online and in-store. This is done by ensuring that you are dealing with the right cardholder and that the purchase is legitimate.
When it comes to identifying the cardholder, credit card fraud detection relies on authentication techniques such as MFA (multi-factor authentication), 3DS, biometrics, and OTP (one-time passwords).
However, it is also possible to detect credit card fraud by looking at anomalies in the transaction. For instance, an IP address could point to a suspicious geolocation. Similarly, a device with a never-seen configuration of software and hardware could raise red flags.
Depending on the kind of detection tools your company uses, you may answer questions about the cardholder identity and intention in real-time or retroactively. In that sense, credit card fraud detection can be either a payment fraud prevention measure or a way to investigate previous transactions.
How Does Credit Card Fraud Detection Work?
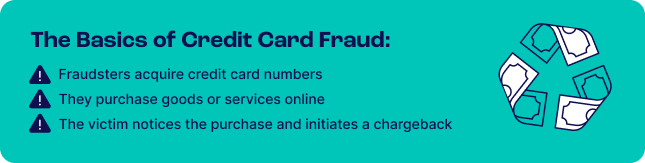
Credit card fraud detection uses advanced technologies, algorithms, and data analysis to identify and prevent fraudulent transactions. Financial institutions employ real-time machine learning models trained to recognize patterns and anomalies indicating potential fraud, such as sudden large purchases abroad or multiple transactions in a short period. The system assesses risk based on transaction history, location, and user behavior. Suspicious transactions may be halted, and the cardholder is often contacted for verification.
In addition to machine learning, credit card fraud detection also relies on rule-based systems, which use predefined rules to identify potential fraud. These rules can include simple conditions like exceeding a spending limit or more complex ones involving correlations between different data points.
Financial institutions use real-time monitoring and behavioral analytics to continuously track cardholder activities. Advanced techniques like biometric verification, tokenization, and multi-factor authentication enhance security. Integrating these technologies allows credit card companies to effectively detect and prevent fraud, protecting both cardholders and financial institutions.
The fraud management industry is set to grow to USD 38.2 billion by 2025, and there are options aplenty. Read our list of the best fraud detection tools to help you today!
Read More
How Do Fraudsters Get Credit Card Numbers?
It’s easier and cheaper than you might think to acquire credit card numbers online. There are thousands of dedicated marketplaces both on the clearnet and darknet. In fact, a report by The Guardian claims you may find prices as low as $17 per card. Here is how they become available:
- Theft: criminals steal or gain access to physical cards and use them.
- Skimming and cloning: making unauthorized copies of credit card details with special equipment known as a skimmer that can be installed on top of a legitimate card reader. The card numbers are then reused for a cloned card.
- Account takeover: when a fraudster gains unauthorized access to someone else’s account. With a credit card linked to it. The problem is even worse if the account acts as an ewallet (BNPL, crypto or neobank account, for instance)
- Phishing and social engineering: taking advantage of people in order to extract key information. Credit card details may be stolen by sending emails or SMS, or by deploying entire fake online shops.
Infiltrating legitimate online stores: criminals inject scripts on existing online store websites, effectively a form of online skimming, which can be done with sophisticated tools such as MageCart.
How to Detect Credit Card Fraud
Since fraudsters have plenty of ways to acquire credit card details, how can businesses know when these details have been stolen? With the following tools and techniques.
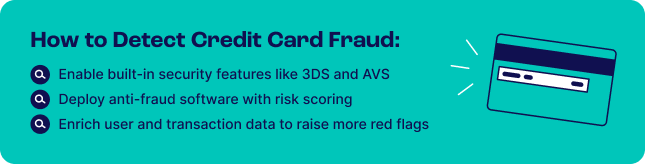
Card Security Features
Credit card networks have developed a number of security features designed to prevent fraudulent purchases. These include:
- Address Verification Service (AVS): A service designed to confirm the cardholder’s identity by looking at their registered address. The address is confirmed against the bank’s records.
- 3-D Secure (3DS): A security layer that prompts users to enter a code to complete a purchase. Different card operators offer the service under different names, such as Visa Secure (Visa), SecureCode (Mastercard), or SafeKey (American Express).
- CVV: A CVV, or Card Verification Value, is a three-digit number located on the card. It is designed to verify that the card is indeed in possession of the customer at the time of purchase.
It’s worth noting that these card security features add a certain level of friction. This is why Amazon, for instance, doesn’t ask for a CVV at the checkout stage, as the company has determined that it slows down the process, impacts the customer experience negatively, and has other defenses in place to make sure it is in fact you logging in.
Risk Scoring
Risk scoring / fraud scoring is a standard risk management method, which uses rules to gauge risk. They help people make educated guesses about a certain user action. For instance, you can use a risk score to determine whether a payment should be allowed on your site or not.
For credit card fraud detection, risk scoring tends to rely on heuristic rules, also known as heuristics. They are shortcuts designed to deliver quick decisions using if-then logic. For example:
- If the IP address points to a different location from the shipping address, then the risk score should go up by 1 point.
When the risk score reaches a certain threshold, an automated system can decide to block or allow the transaction.
A more advanced form of risk rule is called a velocity rule, which looks at data points within a certain time frame to score human behavior. For instance:
- If the user fails to enter the right password five times within one minute, then the account should be temporarily blocked.
By combining multiple risk rules, you can create decision trees that allow for more accuracy in the scoring system.
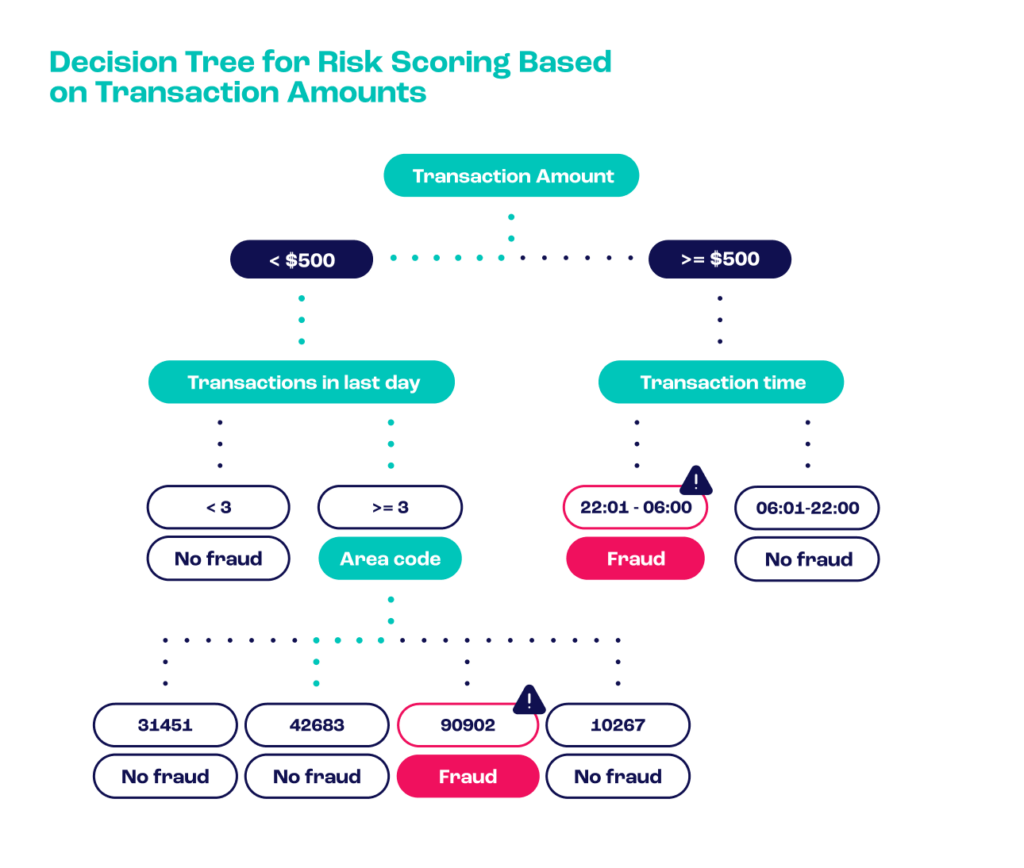
Note that risk scoring may be transparent or opaque. That is to say, risk managers can control and customize the rules, or rely on preset algorithms. The former is referred to as a whitebox system, the latter is called a blackbox system.
Whether you prefer a whitebox or blackbox system depends on your ability to monitor credit card detection.
Companies with fewer resources may prefer relying on an out-of-the-box solution. Those with a dedicated risk management team tend to favor whitebox systems, as they allow for more customization and flexibility.
You can read more about risk rules and best practices in our post on card not present fraud prevention.
Data Enrichment
How do you confirm someone’s online identity before a transaction? You could ask them to submit ID documents. You could use video verification. But is it really worth it for a low-value transaction?
This is the key challenge faced by companies who need to detect fraudulent credit card payments: verifying customers without increasing friction. Too many obstacles between customers and their purchases will create churn, and shoppers will turn to your competitors.
This is why data enrichment is one of the most exciting and effective ways to confirm an identity. It’s an invisible security layer that works by getting more information from a single data point. For instance:
- Device fingerprinting: Learn if the user has connected to your site with the same device in the past. Are they attempting to spoof their connection details?
- IP analysis: Does the connection come from a VPN, a suspicious proxy or Tor node?
- BIN lookup: Is the payment card the right kind? Would it make sense for a customer in APAC to have a prepaid card, for example?
- Reverse social media lookup: Has the phone number or email address been used to register to a social media website? Does the user bio seem consistent with the transaction details?
The main point is to build a user profile without asking the customer for extra information. You can then feed all this data to your risk scoring system, which will help you determine if you are likely to be dealing with credit card fraud or not.
Data enrichment also helps log more information about users. This may come in handy when disputing a chargeback and presenting evidence of friendly fraud, for instance.
How SEON Does Credit Card Fraud Detection
SEON’s fraud detection software features to help with credit card fraud detection. These are designed to give you more information about the payment, cardholder, and their alternative data such as social media presence or device data:
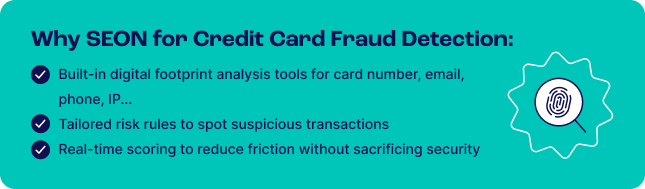
- Card BIN lookup: a powerful feature designed to let you know if the card is valid, which bank issued it, and to which country it points to.
- Social media lookup: enter the shopper’s email address or phone number to learn if they have an online presence. If they don’t, you should investigate further.
- IP analysis: learn everything about how the customer connects to your checkout, and flag harmful IPs, VPNs, Tor usage or suspicious DNS.
- Custom and industry-specific risk rules: SEON comes pre-loaded with risk rule templates tailored to online stores, BNPL or others. These allow you to automate risk management and reduce credit card fraud rates instantly. You can of course customize them to your liking.
- Integration flexibility: leverage SEON in the way that makes the most sense for your credit card fraud challenge. Add an extra layer of data intelligence, connect via API for full automation, or you can even use a plug-in for Shopify.
The key is to deliver powerful tools that help you take control over your credit card fraud rates and reduce chargebacks, without sacrificing friction or security when it comes to accepting payments.
On average, our fraud detection tool helps our customers reduce their fraud rates by 70 to 80%, up to 99%! What can it do for you?
Ask an Expert
FAQ
Fraud prevention software should be able to detect credit card fraud instantly. It will use a combination of risk rules to flag a transaction and prevent it before it happens. If you detect credit card fraud, you will need to initiate a chargeback request. The chargeback may take up to 120 days to be settled.
Theoretically, you can trace credit card fraud. In practice, however, it is very unlikely that the fraudster will be prosecuted – unless they are caught as part of a large-scale anti-fraud operation.
If your business processed a fraudulent credit card transaction, you are legally forced to repay the sum to the cardholder. You may also dispute the chargeback process, which may be a long and costly process.
Credit card fraud happens when a fraudster gets hold of someone else’s credit card details and makes a purchase with it. This is clear fraud, where the goal is to not pay for a good or service and still receive it.
Note that there is also another type of credit card fraud that happens when the cardholder is being dishonest. In that scenario, the payment looks legitimate, but the cardholder has already decided to return the item or ask for a refund.
Sources
- Juniper Research: Online Payment Fraud
- The Guardian: Stolen credit card details available for £1 each online
- Nilson Report: Card Fraud Losses Dip to $28.58 Billion
- Magecart: A Deep Dive Into Magecart