Worried a poker bot farm is hiding on your iGaming site? Use these tools to protect your business and players
Summer 2020 made history in the iGaming community, as a data leak exposed one of the biggest poker bot rings ever found. And the numbers are staggering, even when the dump only looked at a fraction of the operations:
- 60,000 sessions played
- 50 poker sites targeted
- $675,000 earned in rakeback
- $3M earned in winnings
It was a remarkably sophisticated attack, and understandably, players were furious. Had they been losing money to an organized criminal network? Were those online poker sites’ security too lax?
And how should you deal with poker bot farms before they create a PR crisis for your iGaming platform? All the answers below.
What Is a Poker Bot Farm?
A poker bot farm is a term that describes any group of malicious poker bots coordinating to commit poker-related fraud – usually to the detriment of online poker rooms but also, sometimes, of legitimate poker players. These bots collude to fix online poker games for various purposes, from money laundering to bonus abuse.
On its own, a poker bot can help fraudsters cheat the system so they can win or lose a hand of online poker, no matter their opponents’ strategy or luck. When a criminal employs a group of online poker bots, they are able to:
- scale up their efforts, winning more or losing more at will
- fix entire tournaments to win otherwise difficult-to-win prizes
- play through any sign-up or other bonus requirements, thus scamming the poker platform
- collude to conduct money laundering via the poker platform
The Attraction of Online Poker Tables
iGaming, casinos and sports betting attracts all kinds of fraudsters for bonus abuse, account takeover, credential stuffing, and transaction fraud, to name but a few. The quick access to money makes them a high target, as they essentially act as online wallets.
But poker tables and poker sites are the most frequently targeted games, and for two good reasons. Firstly, fraudsters can increasingly deploy software, or bots, that automate online play. These still require some oversight, which is why all the sessions logged in the data dump were from suspicious users who operated between 9-5. They were probably monitored by fraudsters who took it as a job.
Secondly, the fact that poker is a multiplayer game adds another layer of complexity in the form of player collusion.
Put simply, it’s a coordinated effort between players to consolidate a win by having other players lose on purpose, and it’s a huge problem for a variety of reasons. In fact, it’s such a huge problem that online casinos won’t even offer signup bonuses for their poker games.
Partner with SEON to easily spot poker bots & reduce fraud in your business with real-time data enrichment, whitebox machine learning, and advanced APIs.
Ask an Expert
The Rise of AI-Driven Poker Software
Everybody is familiar with the high stake games between human chess players and machines, but advances in artificial intelligence and machine learning mean that researchers are increasingly turning towards more complex games like poker.
As poker combines mathematical skills with human traits like psychology and deception, it was previously thought impossible to learn for computers.
But the University of Alberta Computer Poker Research Group in Canada, for instance, is at the forefront of AI-driven software that can replicate poker player behavior. As of 2020, the group has released five free, open-source programs which attempt to “solve” different poker games, and make them unbeatable.
The problem? The code is fully open source. The program Polaris, for instance, designed to play Texas hold’em poker, is extremely popular for deploying poker bots.
While these tend to play on the lowest limits, even tournament users cannot rule out that they are playing against bots, especially during the middle and late phases, which are strongly influenced by a player’s mathematical skills.
And as technology continues to evolve, it will become harder than ever to differentiate between human players and machines.
When Fraudsters Organize
In a normal multiplayer poker tournament, players are unable to communicate with each other. The practice is called Ghosting, and it is not only hard to pull off, but also prohibited.
However, in the online world, it’s nearly impossible to enforce a policy that prevents players from sharing advice.
And if the players had planned to work together beforehand, in the form of collusion, it becomes even more challenging to spot connections between the players. This creates all kinds of risks:
- Loss of player confidence: The poker economy is a pyramid, where the money rises up to the top 1% and the rest is eaten up by rake or goes to the house. Operators recognize this and do what they can to protect the middle and bottom of the pyramid in feeling safe and that they have a chance to win. But when bots are involved, that trust is eroded and players will move to other operators’ tables.
- Negative PR: as we’ve seen from the backlash against operators whose tables had been infiltrated by bots, players will turn on the company for not doing enough.
- Money laundering risk: organized crime syndicates can use poker tables on iGaming sites to launder their money as a part of an AML fraud, which ends up in potential huge AML fines for your company.
- Expensive damage control: not only will your players migrate to other sites with better reputations, you will also be flooded with a request for refunds. Whether you return the lost funds or not, the damage has already been done as your support team will be overwhelmed, and your reputation hurt.
How SEON Can Detect a Poker Bot Farm
The SEON anti-fraud platform is exceptional at spotting hidden customer connections, stopping multi-accounting and easily finding spoofed devices or locations – the type of tool used by poker botnets to enable their schemes.
Today, we’ll look specifically at the data found in that huge 44GB data set dump from August 2020, and demonstrate how SEON could have helped expose the bots.
First, let’s examine exactly how the poker bot farm ring worked in that particular scenario:
- The fraudsters somehow found players willing to sell their accounts and personal information. These included passport photos, address verification ad bank statements. It’s worth noting that these were real people, not synthetic IDs as in the example of synthetic identity fraud.
- Virtual machines (VM) were created, and IP and device information was faked so that the connections looked like they were from areas near each player.
- Each VM was loaded with all the necessary information including ID data, poker software, payment details. They then create a new account for each identity.
- A fraudster “employee” came into work every day and launches the programs. He also took screenshots and ensured the bots functioned well.
Note that steps 1, 2 and 3 above all relate to the onboarding or registration stage. Here are the verification checks we should apply:
- IP analysis: The fraudsters used proxies designed to spoof IP addresses. This will identify suspicious discrepancies as well as some spoofing tools such as Tor. Risky countries can also be flagged – Russia or Ukraine, for example.
You can SEON’s IP address check below to see the wealth of information it can offer, complete with data points that can point to red flags.
- Device fingerprinting: This particular group of fraudsters had access to spoofing technology designed to trick analysis tools. SEON is able to identify and point out a range of tools used by fraudsters, including spoofing software and virtual machines.
- Email analysis: By analyzing all customers’ email addresses at the onboarding stage, SEON would have spotted that the email addresses used by the fraud ring that ran the poker bot farm all followed similar patterns.
This is most certainly where SEON would have alerted your company of the risk. Most of these players had similar email addresses, such as [email protected], with a few numbers thrown in for good measure.
SEON’s string analysis feature, for instance, can examine an email handle to ensure it’s not too generic, or to compare it with other recent signups. We also check what kind of domain is used, and whether it has been part of a data breach – a data breach lowers the risk score, as it is a sign that you’re dealing with a mature email address rather than one created a few days ago.
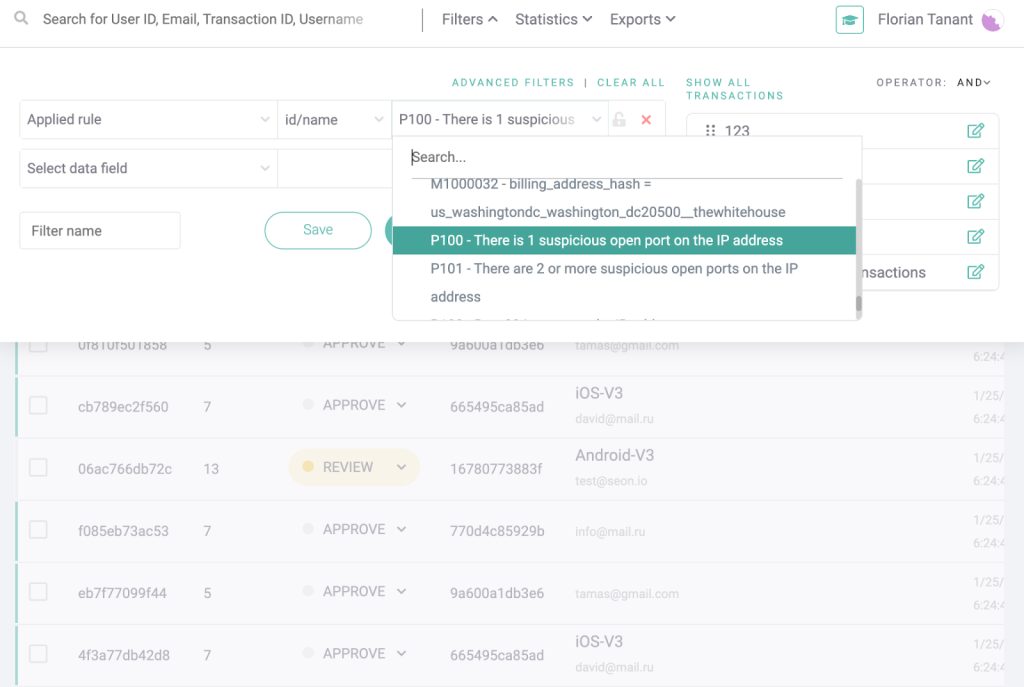
- Phone analysis: Had the fraudsters created virtual phone numbers, SEON would have immediately increased the fraud risk after performing a reverse phone lookup.
- Social media lookup: At SEON, this is a key part of what SEON likes to call digital footprint analysis. Using someone’s email, we search 90+ social media and crowdsourced platforms to see if it has any presence there – from LinkedIn and Twitter to Airbnb and Disney+. Because these addresses were created to enable multi accounting, we would have been able to clearly see that few of the fraudsters had online accounts, which is a red flag.
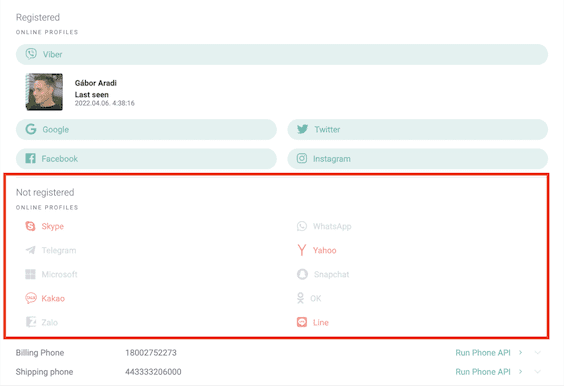
- Velocity rules: Velocity rules attempt to understand player behavior by considering when and how often an action is done. This is a type of rule that can be tweaked at will on the SEON platform – which also comes with industry-specific presets and machine learning suggestions.
For instance, SEON’s velocity rules can identify when users have similarities that could imply they’re the same person – a key pain point for all iGaming brands, poker-related or not. Examples of this include monitoring the time frame between the first and last game, and betting strategies.
In this particular instance we’re studying, he bots ran 9–5 Monday to Friday with consistency, which could have alerted the victims if it had been monitored. Moreover, most of the bot accounts were found on tables with stakes between 10NL and 200NL. Other technical statistics such as post-flop Agg% and comparing stats with banned bots can also be monitored, per the platform’s risk tolerance.
It’s worth highlighting that SEON’s granular, customizable software lets you create custom fields specifically to analyze this kind of data, which wouldn’t be taken into account by a standard transaction fraud solution.
Partner with SEON to reduce fraud in your business with real-time data enrichment, whitebox machine learning, and advanced APIs.
Ask an Expert
Don’t Let Poker Bots Hurt Your Reputation
A poker bot farm by itself doesn’t necessarily hurt your operations. In fact, shadier iGaming companies have been known to populate their games with bots alongside real players. Similarly, the best poker players have no problem playing against them.
The issue, however, is that the majority of your players will feel cheated if a farm operates on your platform. And as leaks from 2015 and 2020 show, players will take matters into their own hands to find suspicious behavior, and will complain to you directly.
In other cases, there’s more than your reputation that you have to lose, as poker bots can cost you your AML compliance, as well as some of the bonuses you are wanting to hand out to legitimate customers.
Luckily, even the most sophisticated bot rings can be spotted with a combination of digital footprint analysis, device fingerprinting, velocity checks and IP analysis at the point of registration and powerful custom rules created by your fraud managers and the machine learning module.
You might also be interested in reading about:
- SEON: How to Prevent iGaming Fraud
- SEON: Reducing Bonus Abuse in iGaming
- SEON: Stop Multi Accounting Fraud
Learn more about:
Digital Footprinting | Browser Fingerprinting | Device Fingerprinting | Fraud Detection with Machine Learning & AI
Sources
- VIP Grinders: MASSIVE POKER BOT FARM DETECTED ACROSS MULTIPLE ONLINE POKER SITES
- University of Alberta: University of Alberta Computer Poker Research Group