11 Types of Telecommunications Fraud: How to Detect & Prevent It
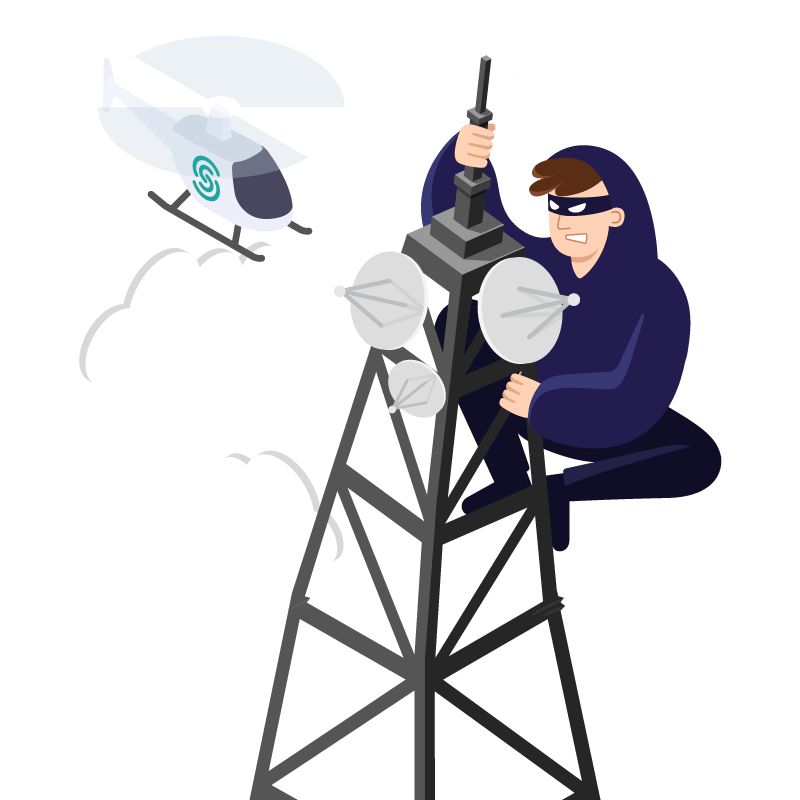
by Jimmy Fong
With sources expecting the global buy now, pay later (BNPL) market to grow over 20% year-on-year, BNPL providers have their hands full, and not just with money but with potential BNPL Fraud. This growth rate may even be on the conservative side, considering that Apple has just started inviting iOS 16.4 users to it’s hot-off-the-presses Apple Pay Later microlending service, backed by Mastercard and Goldman Sachs.
This new player in the BNPL game will surely give other major BNPL microlenders like Klarna and Afterpay a run for their money — broken into four more affordable sums, of course. Considering this new hat in the ring on top of that projected rate of growth, market data research firms variously estimate that the global BNPL value will balloon to well over $500 billion within the next five years – a number that will inevitably attract the attention of malicious payment fraudsters, as well as regulators.
A BNPL payment ecosystem offers its customers increased buying power. However, as with any unique payment system, it also has unique fraud challenges to deal with. What are those challenges, and how can providers avoid a Fraud Now, Pay Never situation?
Partner with SEON to reduce fraud rates in your business with real-time data enrichment, whitebox machine learning, and advanced APIs.
Ask an Expert
In the pursuit of more sales and new markets, BNPL providers create a payment ecosystem with lower security friction and a larger attack surface. These vulnerabilities appear across the BNPL landscape, from pre-onboarding onwards. The biggest leaks in the ship open up around the low-friction onboarding, extended attack surface, and overall volume and popularity.
First, consider the noble side of what buy now, pay later systems offer: spending power and democratization. With BNPL, where customers can pay for things in installments over several months, items that were unaffordable are suddenly manageable. For retailers, this represents the miraculous discovery of new markets.
The less-than-noble side? BNPLs have been accused of facilitating lifestyles that people can’t afford, but which microloan providers still make money on – indeed, they often make more due to late repayments.
To maximize the user base, BNPLs sand off the hard edges of security friction wherever possible. Though this is not explicitly stated, it seems reasonable to assume that BNPLs want to encourage impulse buying, citing the affordability of just about everything when paid for on an installment plan.
Moments of standard security friction such as, say, filling out a comprehensive financial form that bank-issued loans would insist on, offer the impulse buyer a chance to reconsider: “Do I really need this?”
Criminals see this low-security hurdle and know how trivial it is to jump it, meaning instances of synthetic identity fraud and multi-accounting are harder to find in a timely manner.
BNPL providers are unwillingly but unavoidably providing payment fraudsters with a much larger target to hit. This is because of the nature of buying now and, importantly, paying later. The introduction of additional months to the payment process opens up microloan providers to some very specific pain points:
In this last example, there were multiple points where payment fraud was committed, and the fraudster got away with their illicit purchase. The increased attack surface – in this case, increased over time and multiple payments – necessitates increased security coverage.
The success of companies like Klarna has seen flocks of customers using BNPL services to increase their buying power and access products that were previously unattainable. According to Juniper Research, last year the volume of BNPL shoppers was estimated to be over 360 million worldwide.
In the eyes of the providers, this impressive traffic volume – as well as the profit that goes alongside it – is at least partially due to the low-friction user experience they create. Unlike most loan providers, BNPLs are not currently required to carry out the credit checks associated with best practices in that sector. This can be seen especially during the holiday shopping season, when many BNPLs will make their security checks even lower, to usher in as much of the holiday rush as possible, according to a report published by CNBC.
The overall popularity of BNPL financial services, and especially the surge during major shopping seasons, gives scammers the cover of being a needle in a massively lucrative haystack. Businesses may even calculate that the higher profits from lower security may outweigh the benefits of curbing fraud – at least until regulators take notice.
Stopping payment fraud in the BNPL space revolves around risk-based fraud detection as part of a security-focused user onboarding platform, and also at each payment. Though, as discussed, security friction and BNPL tend not to play nicely together (at least according to revenue teams). Microloan providers who want to shore up their defenses against payment fraudsters should be looking for tools that create the most comprehensive customer profile without introducing extra time in which the customer could say to themselves: “Forget it, I shouldn’t anyway…”
To effectively stop the various threats that payment fraudsters might offer, new customer onboarding processes and transaction monitoring protocols must be robust.
A serious effort to prevent ATO attacks that could result in the whole spectrum of retail losses – no product, no payment, chargebacks to the account holder, and so on – should include continuous monitoring of user accounts to detect signs that an unauthorized user has logged in.
To minimize fraudsters creating new accounts and exploiting the “pay later” aspect of BNPL, perhaps only paying the initial 25% and then abandoning the account, fraud teams should make sure they are conducting comprehensive device fingerprinting, IP checks, and digital footprinting. Naturally, these functions require software capable of real time payment transaction monitoring. This effectively stops a single malicious first-party fraudster from casually squeezing the most out of a BNPL microlender.
To implement strategies that close loopholes for payment fraudsters, your BNPL should consider introducing risk-based security rules like these, which are easily integrated with SEON.
A common form of payment fraud in the buy now, pay later ecosystem is the simple never-pays fraud. This is where a customer orders a big-ticket item, triggers the fulfillment of that order by paying the first installment, then vanishes with the delivered item. Fraudsters who have scaling in mind with this scam, for example by flipping Playstation 5s that they only invested 25% of the retail price in, will likely be doing so from a single device.
An easy way to detect this form of pervasive fraud is to scrutinize new customers’ device hashes for suspicious patterns. By labeling instances of never-pays fraud, SEON can scrutinize all new customers for the same device setup. This setup is almost always unique, given the multitude of data points that make up this fingerprint, including things like installed browser language, add-ons, and even the resolution of the user’s screen.
When the same setup as a labeled scammer approaches your domain, it should be flagged, at least for manual review – if not outright blocked. If the apparent new user is, on review by a member of the fraud team, almost certainly the same user as a previous scammer based on their unique device hash, their continued usage of the BNPL system should certainly be prevented.
The above screengrab shows how, within the SEON platform, you can easily set up a custom rule to detect instances of the same device approaching your platform within a particular timeframe. The associated fraud score that this triggers, as well as the time frame and name of the rule, are all fully customizable, and are implemented as soon as the changes are saved.
Though there may be any number of legitimate reasons a BNPL user might suddenly be logging in from a new device in a new location – a new phone, new home, or vacation come to mind – these instances still deserve a double-check from a member of the fraud team to make sure this is not a case of account takeover (ATO). Slightly less suspicious is just a new IP or new device, but if both occur together, the risk is significantly higher.
If not manual review, you can also implement safeguards such as two-factor authentication (2FA) or a follow-up call from customer service to make sure sign-ons like these are not malicious ATO attackers who are hoping to use stored payment credentials to make unauthorized purchases.
In the above screengrab, SEON has been set up to detect exactly such a case. Specifically, the action type is defined as account login, as opposed to a different behavior on the site (such as onboarding or payment), and at that stage, SEON compares the IP and device to historical data for that user. This rule has been set up to add 15 points to the overall risk score, which, depending on your organization’s risk appetite, should probably trigger a manual review.
Fraudsters hoping to take advantage of BNPL by simply never paying will very likely be creating hollow shell accounts if they are hoping to scale their enterprise. To buy multiple TVs or handbags for resale, for example, a first-party fraudster will need a new account for each translation they initiate – and intend never to complete payment on.
While device fingerprinting and IP addresses may suffice to catch some of these scammers, augmenting the onboarding process with an additional custom rule will give fraud teams a more comprehensive profile to make their determinations.
This screengrab shows SEON’s default rules, which flag instances of email accounts associated with no known social media registrations – a suspicious oddity for a trustworthy email account. It shows that the email address itself is very likely new, given that it has not been involved with any known data breaches.
If an onboarding customer triggers this rule, particularly in conjunction with triggering any device fingerprinting rules, the user is very likely to have unscrupulous intentions for your BNPL service, meaning you should treat them as a risk. Though this is a default rule that comes packaged with every SEON account, you can customize all the data points for scrutiny in the rule editor, as shown in the previous two screenshots.
SEON’s advanced device hash analysis easily uncovers multi-account abusers before they can wreak havoc in your domain.
Ask an Expert
Keeping the BNPL space free of fraudsters is an inherently challenging task when compared to a traditional payment ecosystem. As discussed, the ability to buy now and pay later offers an extended attack surface, especially in terms of the timeframe, and operators have a financial motivation to keep security checks low and purchases flowing. Policing this ecosystem requires a solution with a subtle but effective touch, such as SEON.
You can easily set SEON’s custom rules to counteract the typical BNPL payment fraudsters, such as ATO and never-pays scammers. You can adjust those rules in seconds as new patterns or specific pain points emerge in your data. The platform’s machine learning tools will also generate rule recommendations over time, looking for nuanced patterns in the behavior of those taking advantage of the extended attack surface.
In particular, the unsophisticated first-party fraudsters, armed with new email addresses to make many new accounts, are easily foiled by SEON. These fraudsters, who so often beset BNPL providers, should not be able to make it far past the onboarding stage, as their fraudulent profiles will certainly lack the realism of a legitimate, lived-in email address. They will either be obviously from the same location or else show signs of device spoofing – both of which SEON can easily detect.
Showing all with `` tag
Get anti-fraud and compliance insights and tips from SEONs experts.
Jimmy Fong is the Chief Commercial Officer of SEON. His expertise in payments saw him supervise the acquisitions of companies by Ingenico, Visa and American Express. Jimmy’s enthusiasm for transparent sales and Product-Led-Growth companies drives SEON’s global expansion strategy, and he interviews both fraud managers and darknet fraudsters in our podcast to stay on top of the latest risk trends. Yes, it’s also him wearing the bear suit on our YouTube channel.