Alternative credit scoring evaluates creditworthiness beyond traditional credit reports and scores, such as those by Experian, Equifax and TransUnion, the three global credit reporting agencies. According to the Consumer Financial Protection Bureau (CFPB), approximately 26 million Americans are “credit invisible,” meaning they have no credit history with a nationwide consumer reporting agency. Additionally, 19 million Americans have insufficient credit histories to generate a credit score, making it difficult for these individuals to access credit. This only looks at the US as a microcosm of the more significant issue.
Globally, 1.4 billion adults are unbanked, and in low and middle-income countries, fewer than 10% of individuals can be found in public credit registries. This means that hundreds of millions of adults worldwide are excluded from the formal financial system and lack meaningful access to credit products like loans or lines of credit. Such a pronounced gap between “unscorable” people and the need for financing results in a big opportunity for online lenders willing to use alternative methods to evaluate creditworthiness accurately.
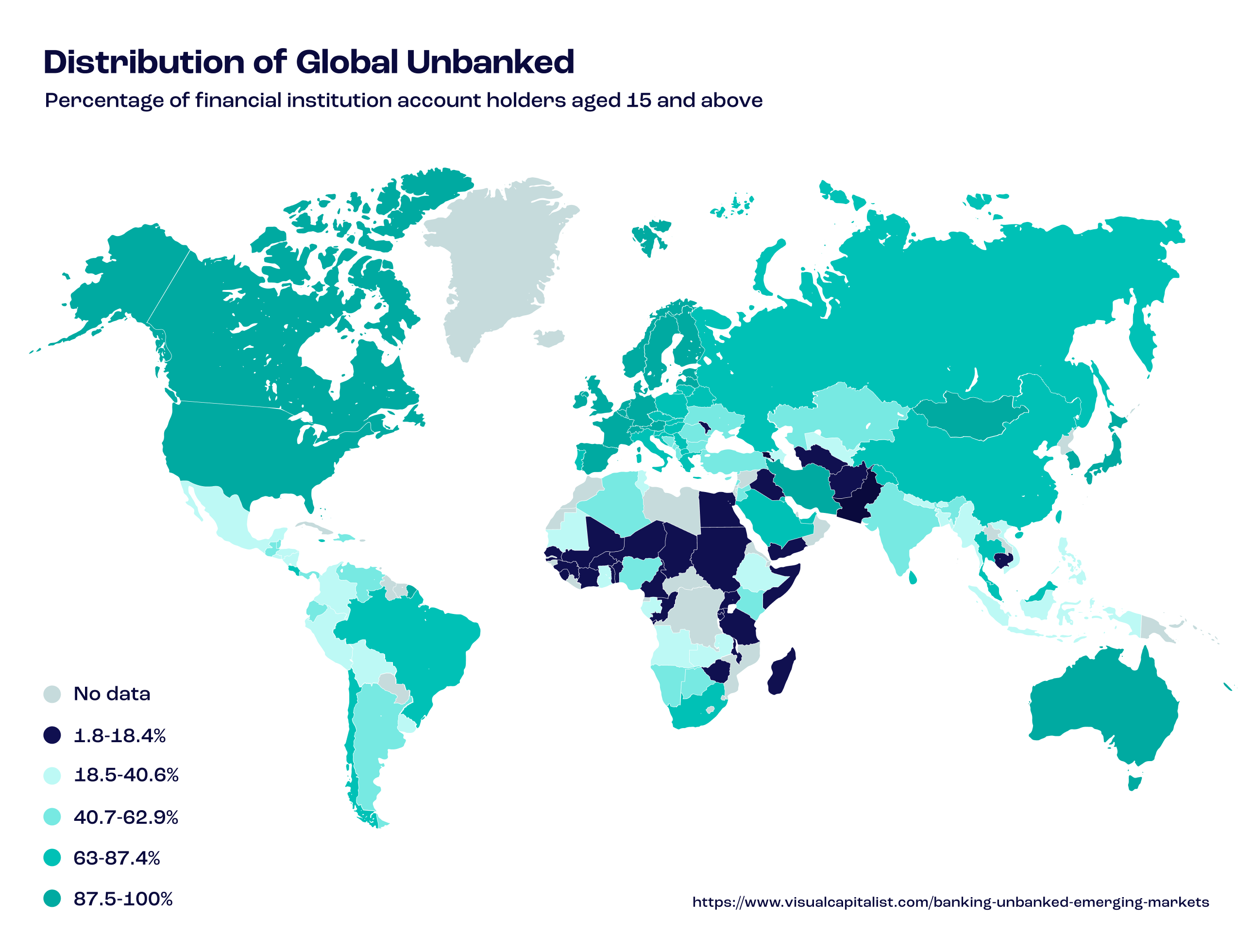
What is Alternative Credit Scoring?
Alternative credit scoring is used to examine additional data sources and other relevant factors to assess borrowers with limited credit histories or who are categorized as “unscorable” by today’s conventional models. While effective, traditional models exclude millions of consumers with insufficient credit data – including young adults, immigrants, women in rural areas and those who prefer cash transactions or live in cash-based economies.
For lenders in unbanked and underbanked regions, institutions that want to engage with younger generations with mistrust for big banks or traditional credit lines or individuals who simply don’t have access to the traditional financial system, there are certain obstacles to assessing creditworthiness outside conventional financial services. This is where alternative credit scoring, a modern method representing an evolution in risk scoring for lending institutions and firms, can be a game changer.
Evaluating Additional Alternative Credit Signals
To create a comprehensive assessment of a borrower’s creditworthiness, various signals serve to determine whether an applicant has the means and the intention to repay a loan. For example:
- Utility and Rent Payments – Timely payments for utilities, rent and phone bills can demonstrate financial responsibility.
- Bank Account Information – Analysis of bank account activities, including deposits, withdrawals and savings patterns, provides insight into a borrower’s financial stability.
- Employment and Income Data – Steady employment and consistent income are strong indicators of a borrower’s ability to repay debts. Employment data can also be combined with sector data, allowing the lender to estimate a debtor’s income level to make a suitable loan offer.
- Education Background – Higher education levels can correlate with better financial habits and higher earning potential.
- Social Media Activity – In some cases, social media behavior and connections can offer additional context about a borrower’s lifestyle and reliability. For example, a LinkedIn account and its details can suggest that an applicant is a natural person in a regular employment situation.
- Transaction Data – Detailed transaction histories from digital wallets and payment apps can reveal spending habits and financial discipline.
- Online Data Points – Additional data points can reflect a higher level of ‘lending trust.’ For example, suppose a customer regularly pays their bills to several online accounts, such as Netflix and Amazon Prime. In this case, their behaviors suggest that these customers have the finances to repay small loans.
- Digital Footprint Analysis – Information from an individual’s digital presence and activities, including a broad spectrum of social signals.
- Device Intelligence – Process of looking at unique device identifiers based on attributes and configurations.
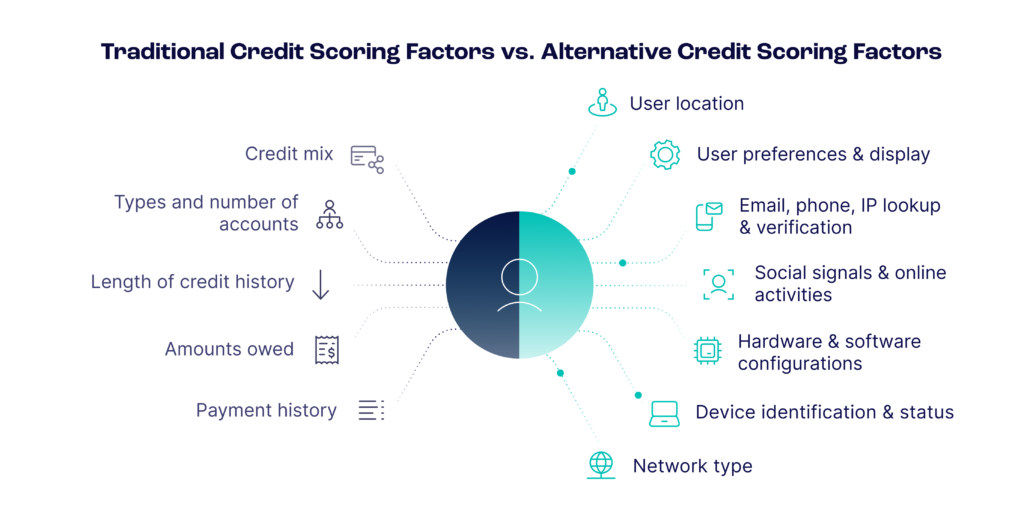
The Digital Footprint as an Alternative Signal
Digital footprint analysis, also called digital footprint monitoring or assessment, involves collecting and analyzing data from an individual’s online presence or activities and various real-time digital, social and behavioral indicators. This type of information gathering simplifies the process of verifying customer information, helping financial institutions to examine alternative indicators of economic stability while enhancing fraud prevention to enable organizations to distinguish between legitimate, eligible applicants and those who are ineligible or just attempting deception.
Digital footprint analysis is handy in markets where traditional bureau data is unavailable, other data sources are stale or inaccurate or the target group or individual is simply underbanked – all situations that make it difficult for lending organizations to assess risk meaningfully. By providing a deep and data-driven understanding of a user’s digital behavior, lenders can leverage these profound insights into risk assessment to better determine the likelihood of financial affordability for any given customer with great precision.
How to Collect Alternative Data for Credit Scoring
Beyond profiling a customer with digital footprint analysis and looking at the above alternative credit signals, additional data can be collected to verify a potential lending customer’s integrity and validity and evaluate further creditworthiness through device intelligence.
Device intelligence represents a sophisticated approach to understanding and analyzing potential borrowers’ technological behaviors. This method involves collecting and examining data points related to individuals’ devices, such as smartphones, tablets and computers. By scrutinizing hardware and software configurations and user interactions with these devices, lenders can gain deeper insights into an applicant’s identity and financial behaviors.Unlike device fingerprinting, which focuses on creating unique identifiers based on device attributes, device intelligence analyzes behavioral patterns and contextual data. This comprehensive analysis helps identify anomalies that might indicate fraudulent activities. For example, unusual login attempts from different locations within a short time frame or multiple transactions from a single device using different accounts can be red flags. Through continuous monitoring and dynamic data analysis, device intelligence helps lenders differentiate between legitimate users and potential fraudsters, thereby enhancing the accuracy of credit assessments.
Practical Applications of Device Intelligence in Credit Scoring
Device intelligence can be particularly effective in regions with high fraud rates or where traditional data sources are unreliable. Lenders can build a robust profile of a borrower’s credibility by leveraging real-time data points, like geolocation, usage patterns and network information. For instance, a borrower consistently accessing their account from the same device and location is more likely to be trustworthy than one showing erratic device behavior.
Additionally, integrating device intelligence with other alternative credit signals, like bank transaction data and digital footprint analysis, provides a multi-faceted view of the borrower’s financial stability and intentions. Together, this view helps prevent fraud and ensures that deserving borrowers who are out of reach of traditional credit scoring models get fair access to credit. By incorporating device intelligence into the credit scoring process, lenders can make better choices, reduce default risks and foster financial inclusion for a broader range of consumers.
Looking at Digital Lending and Risk: Loans Default Vs. Fraud
The rise of digital lending has revolutionized the financial industry by making it easier and faster for borrowers to access funds. However, it has also introduced new risks for lenders. While legitimate borrowers benefit from the ability to create new accounts and access their money quickly, the same ease of access opens the door to individuals likely to default on loan repayments or take out loans under fraudulent premises.
Microfinance institutions today face significant risks from loan defaulters and fraudsters who intentionally target loan offers with no intention of repaying. This poses a considerable challenge as the losses from fraudulent digital loans are much higher than those in other industries—with 7-8% of revenue lost by fintechs in 2021 and 2022. Therefore, microlenders must distinguish between genuine cases of loan default, where borrowers cannot repay due to financial difficulties, and intentional fraud, where borrowers deliberately deceive lenders to obtain funds with no plan to repay.
Current loan fraud tactics involve deceptive tactics such as borrowers submitting falsified documents or stolen identities (third-party fraud) to secure a loan. In second-party or straw buyer fraud, fraudsters collude with a willing second person to use their information for loan approval. Additionally, borrowers may provide false information about their financial situation and other relevant details to secure better loan terms.
The impacts of loan fraud can be severe. Even a small number of malicious applicants can undermine the reputation and efforts of legitimate microfinance institutions. To counter this, looking at alternative signals and employing technological solutions—such as enhanced screening processes with machine learning algorithms, real-time monitoring and creating a comprehensive profile of an applicant’s digital behavior and practices—can improve fraud identification processes and aid in protecting lenders from the losses associated with fraudulent digital loans.
See how to leverage digital footprinting to gain a comprehensive view of financial reliability to enhance decision-making.
Read More
The Benefits of Alternative Credit Scoring
One of the primary benefits of alternative credit scoring is its inclusivity. By considering a broader range of data sources, lenders can extend credit access to individuals often excluded through traditional models. By incorporating non-traditional data, like utility and rent payments, employment history and educational backgrounds, these individuals can demonstrate financial responsibility and access previously unavailable credit opportunities.
Another critical advantage is accuracy. Whereas traditional credit scores rely heavily on a narrow set of financial behaviors, primarily focused on credit card usage and loan repayments, alternative credit scoring integrates diverse data sources, providing a comprehensive view of a borrower’s economic behavior. This holistic approach allows for a more accurate risk assessment, capturing nuances in financial habits that traditional models might overlook. For instance, consistent payment of rent and utilities, steady income and responsible management of bank accounts can all indicate a borrower’s reliability, even if they lack extensive credit card history.
Personalization is another critical benefit of alternative credit scoring models. Through this route, lending models can be tailored to specific demographics or sectors, offering a more customized (and nuanced) approach to credit evaluation. By focusing on the unique financial behaviors and the needs of different groups, lenders can make more informed decisions and offer products that better fit the circumstances of various borrowers.
Innovation in the form of advanced technologies such as artificial intelligence (AI) and machine learning (ML) heralds significant benefits for credit scoring. These technologies support the continuous improvement and refinement of credit scoring models, able to analyze vast amounts of data at high speed to identify patterns and trends that would be impossible for humans to detect. By allowing credit scoring models to learn and adapt over time and improving their predictive accuracy, these technologies make alternative credit scoring more efficient and effective in identifying and mitigating potential risks.
Lending is All About Trust
Building a solid and efficient online credit scoring model in the digital era is challenging. The good news is that SEON’s digital footprint analysis and device intelligence can lower default ratios without closing the door to valuable customers.
At its core, lending is founded on trust, the confidence that a borrower will commit to repaying the funds they’ve been provided. However, determining trustworthiness is complex, especially when assessing individuals with limited financial histories or those operating outside of traditional banking systems. The key lies in finding the right signals that allow lenders to accurately evaluate a customer’s credibility and intent.
By thoughtfully incorporating alternative credit scoring models, lenders can make more informed decisions, extending credit opportunities to underbanked or unbanked populations that may appear excessively risky based on traditional metrics. By harnessing the wealth of social and digital signals, lenders can promote financial inclusion while effectively managing risk—a win-win scenario for businesses and consumers.
Sources
- Consumer Financial Protection Bureau: “CFPB Explores Impact of Alternative Data on Credit Access for Consumers Who Are Credit Invisible”
- FDIC – How America Banks: Household Use of Banking and Financial Services
- Investopedia: Default RiskL Definition, Types, and Ways to Measure
- American Banker: Business lending fraud still higher than before COVID, survey says