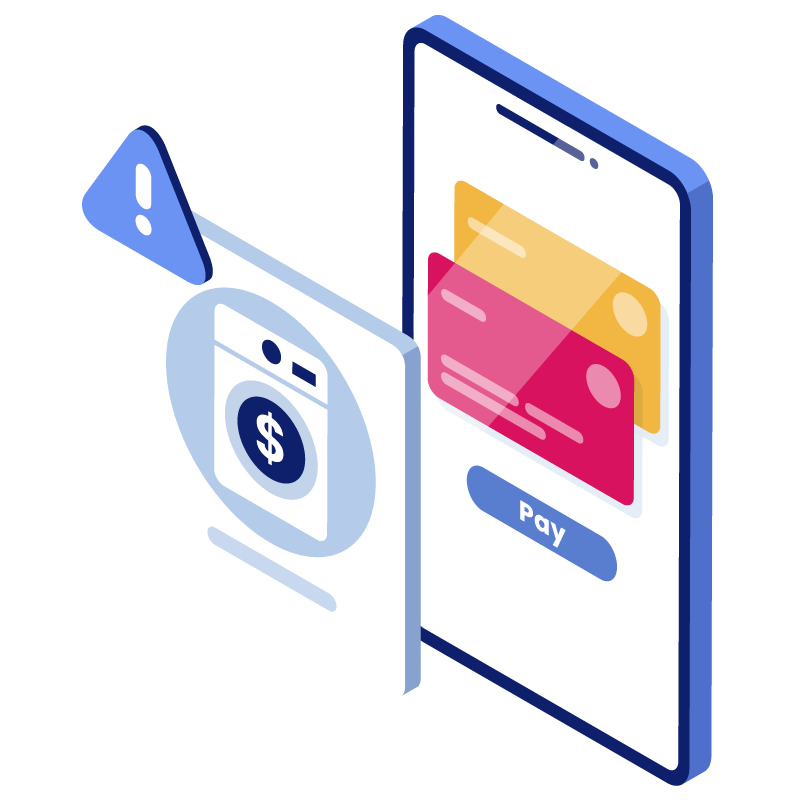
Money Laundering Detection for Digital Banks

Last Updated: May 6, 2024 by PJ Rohall
Amid the digital revolution, where the boundaries of traditional banking dissolve into the virtual world, money laundering poses a significant challenge for digital banks, and navigating compliance is just one piece of the puzzle. It is a global epidemic that transcends geographical confines and jurisdictional boundaries.
According to the United Nations Office on Drugs and Crime, an estimated $800 billion to $2 trillion — equivalent to 2-5% of global GDP — is laundered annually, fueling organized crime, terrorism, and corruption on a monumental scale.
The challenge of detecting and preventing money laundering has reached unprecedented levels of complexity. As traditional financial institutions increasingly digitize their operations and new fintech players enter the market, the need for robust anti-money laundering measures has never been more pressing.
In this guide, you can discover how to boost your Anti-Money Laundering efforts without adding friction to the journey.
Why Is Money Laundering a Problem for Digital Banks?
The United Nations estimates that a whopping $2 trillion of dirty money ends up laundered by banks every year. Some of it will inevitably go through neobanks and digital banks.
This inevitably results in compliance issues, hefty fines and, at worst, reputational damage.
On the other side of the spectrum, having too strict AML fraud prevention software in place leads to false positives, which upsets customers and also damages your reputation.
How can you strike a balance between seamless user experience and safety?
How Can Digital Banks Detect Money Laundering?
Detecting money laundering is a strictly regulated process for banks, which includes:
- Transaction monitoring: This involves keeping a close watch on transactions above a certain threshold. You may also want to look at how regular the transactions are, and where the inbound and outbound funds come and go.
- Real-time alerts: When a transaction threshold is met, you should immediately make a note of it via an automated alert system.
- KYC and ID validation tools: You should combine your KYC and AML steps to confirm your customers’ IDs at the onboarding stage.
- PEP and sanction list checks: Another important check at the onboarding stage involves looking at whether your customers are at risk of financial corruption (PEP) or based in countries flagged for money laundering (sanctions lists).
Top 3 Custom Rules for Money Laundering Detection in Digital Banks
SEON allows banks to get AML watchlist results from PEP and RCA lists, sanctions lists, crime watchlists, etc, and run these checks regularly to contribute toward your AML compliance program.
Custom rules set up on the SEON platform will also help you detect and thwart attempts at money laundering. Let’s now look at some examples of risk rules you can set up to fortify your AML efforts.
#1: IP Address from a Sanctioned Country
One of the most important AML rules is also extremely simple to set up. It’s all about understanding whether you are dealing with a user in a sanctioned country or not.
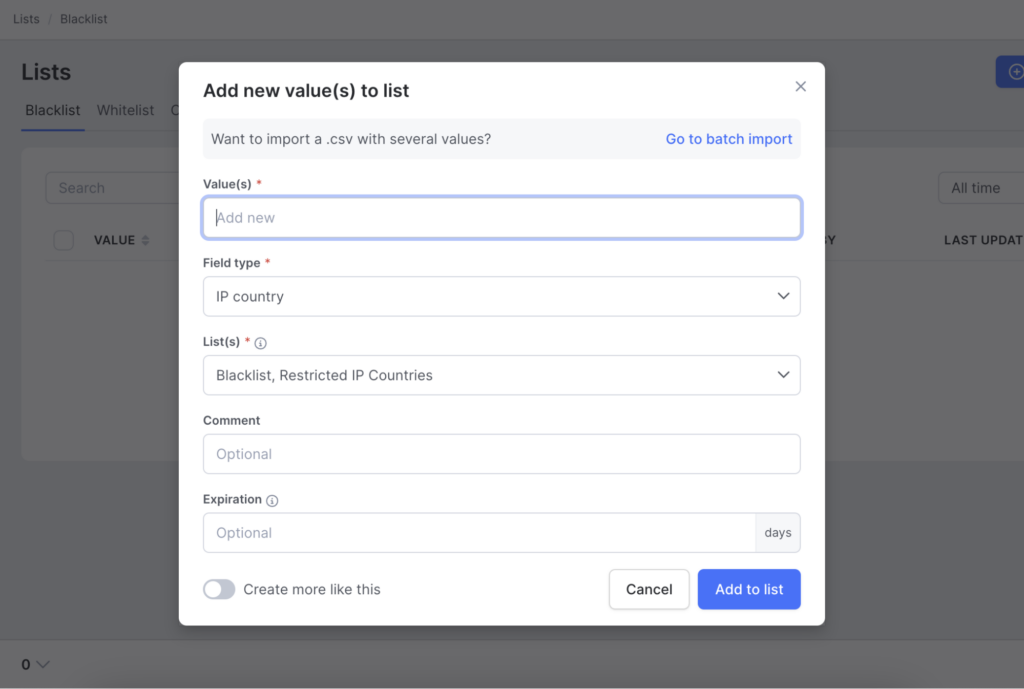
This is a great solution to deploy at the onboarding stage to filter out suspicious signups instantly.
You can easily blacklist IP countries or add them to custom lists, which means that any time a user enters your platform, SEON will check for their IP address and location and, based on this information, make an automated decision on either blocking them, letting them move along or sending the user action for further investigation.
Of course, you can take control over what happens after that flag is raised and exactly which countries will set off the alarm. Most digital banks will send the suspicious transaction or person for review by their manual team, but you can also approve it and attach a note to the customer profile.
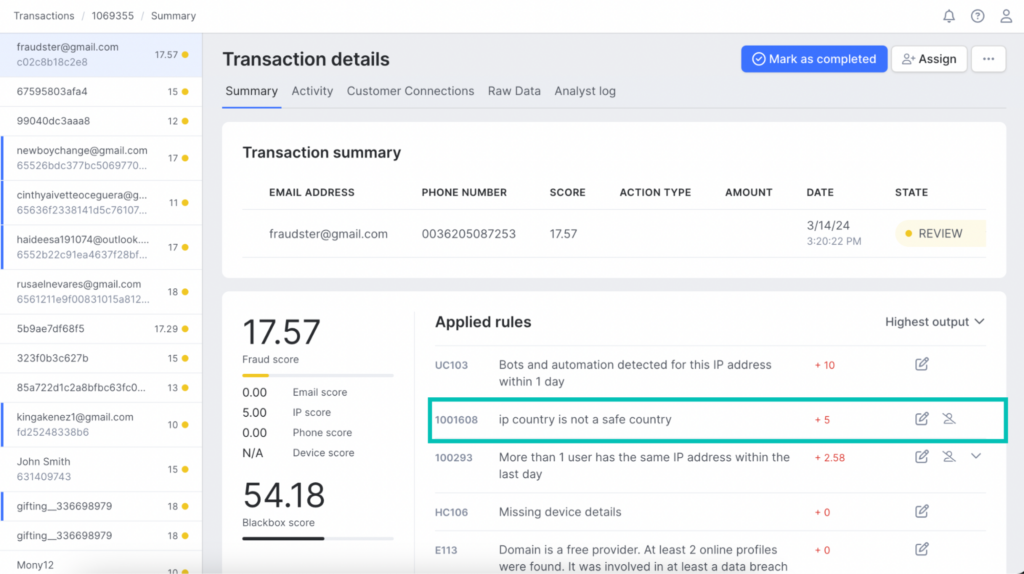
#2: Unusually Large Transaction
AML transaction thresholds are regulated by government bodies. In the US, for instance, you must mark any transaction above $3,000. In the UK, the limit is currently set at £8,800.
This is a key feature of most AML software, and here’s what it would look like as a risk rule in SEON:
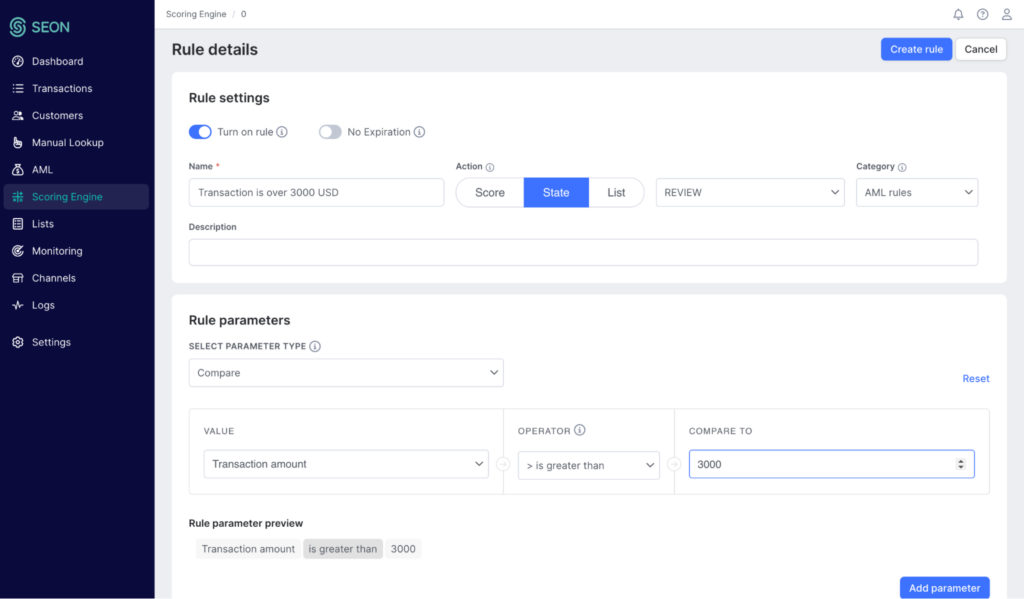
We can clearly see that if the transaction amount exceeds 3,000 (you can specify the currency in your settings), the transaction will immediately be flagged for review.
#3: Increase in Transactions Over a Set Period
Velocity rules, also known as velocity checks, come in handy when you want to get alerted if there’s a sudden increase in the number or value of transactions over a short time period, which is also a typical signal of potential money laundering activity.
These rules allow you to look at data over a set period of time – for instance, an aggregate of all the previous transactions made by one person. You can then compare the value to their latest transaction. See a sharp increase? Time to flag it.
This velocity rule below would add 20 points to the risk score.
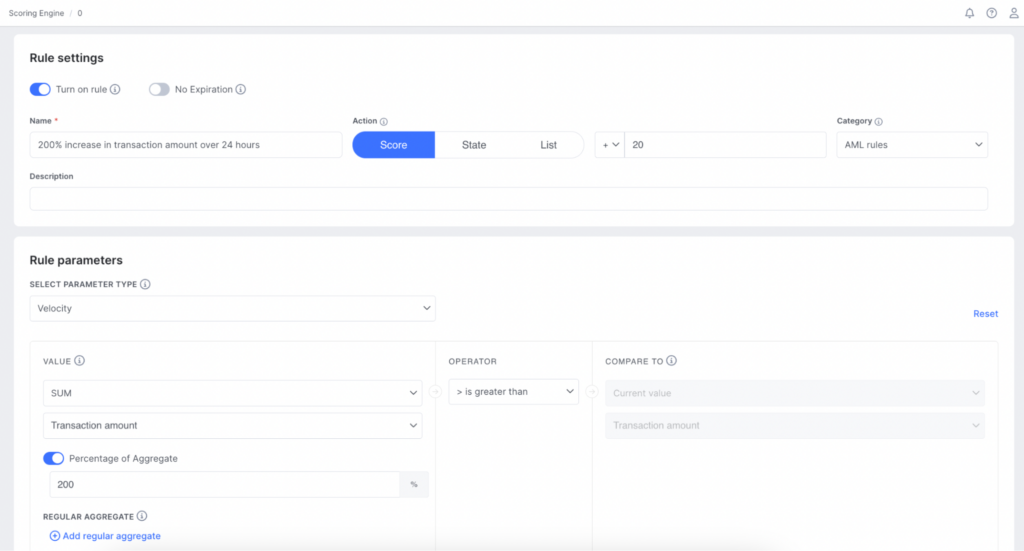
Note that just like you can look for anomalies, you can also attempt to find extremely regular payments.
In fact, as part of your AML strategy, it is highly recommended to identify users who regularly and frequently pay the same amounts into and out of their digital bank accounts.
Partner with SEON to reduce fraud in your business with real-time data enrichment, whitebox machine learning, and advanced APIs.
Ask an Expert
How SEON Can Help in Money Laundering Detection
Digital banks and neobanks turn to SEON for comprehensive solutions tailored to their needs.
SEON’s AML screening platform seamlessly integrates with automated transaction monitoring systems. This integration ensures regulatory compliance by swiftly identifying Politically Exposed Persons (PEPs), Relatives or Close Associates (RCAs), matches with sanctions lists, and hits on watchlists. Furthermore, banks can further customize their defenses by creating rules based on SEON’s AML screening results.
SEON’s pre-screening capabilities add another layer of protection by delving into customers’ digital footprints across 90+ social and digital platforms. By analyzing email addresses and phone numbers against social media profiles and other digital traces, SEON enables banks to spot inconsistencies and automatically flag suspicious individuals for further investigation. This proactive approach enhances due diligence measures, enabling banks to identify potential risks before they escalate.
SEON also equips banks with the means to block transactions originating from suspicious devices or connections. Leveraging powerful device intelligence and IP analysis tools, SEON can identify anomalies such as mismatching IP geolocations compared to billing and mailing addresses. By blocking transactions associated with suspicious activities, SEON fortifies banks’ defenses against fraudulent attempts to launder money through their systems.
Frequently Asked Questions
Though it can take many forms, banks can detect money laundering in their systems by adhering to KYC best practices both at onboarding and continually for potentially risks shifts in geolocation. Then, a KYT framework should also be adhered to to detect signs of unusual behavior, such as large, unexpected movements of cash, many small transactions, or else payments and connections which don’t seem to have a viable business purpose. Manual teams, when risk signals like the above are discovered, may then investigate further to uncover more signs of money laundering like unnecessarily complex ownership structures.
Related Case Studies for Digital Banks
- FairMoney Onboards Better Neobank Customers Thanks to Digital & Social Footprint Checks
- Leading Challenger Bank Eradicates Bonus Abuse Thanks to SEON’s Email Module
Related Articles for AML
- What Is AML Transaction Monitoring & How to Set It Up
- Top Anti Money Laundering (AML) Software & Tools 2022
- Understanding AML in Banking & How It Works
Sources
Articles
Showing all with `` tag
AML Compliance Officers: What They Do and Why They’re Crucial
How to Detect Money Laundering in Forex Trading
What Is Layering In Money Laundering & How Does It Work?
Subscribe to our newsletter
Get anti-fraud and compliance insights and tips from SEONs experts.
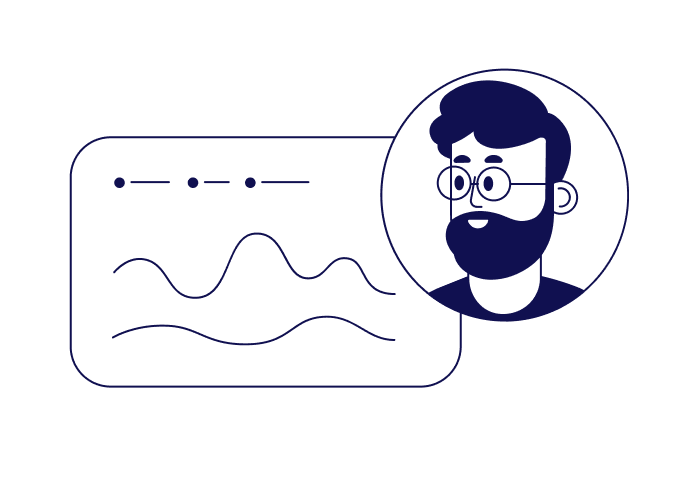
