False positives are the silent killers of customer trust. When fraud prevention systems misfire, they don’t just block fraud — they block legitimate customers as well. That means lost revenue, broken user journeys and rising churn. As fraud threats evolve, so must your defenses. But tightening the net shouldn’t come at the cost of alienating real users.
What Are False Positives?
False positives happen when a fraud prevention system mistakenly flags legitimate behavior as suspicious. That could mean a blocked login, a declined payment or a denied refund request triggered by protective systems misfiring on good users.
Some call it the “customer insult rate” because these events create friction for real customers. When systems are too aggressive, you risk losing conversions, frustrating users and even pushing them toward competitors.
What Is the False Positive Rate?
The false positive rate is the percentage of legitimate actions incorrectly flagged as fraud. For example, if 5 out of 100 flagged actions are actually valid, that’s a 5% false positive rate.
Even a small rate can lead to lost revenue and loss of trust in the brand. The challenge is reducing false positives without weakening your overall fraud defenses.
What Causes False Positives?
If Most false positives stem from overly rigid rules, badly calibrated fraud scores or poorly tuned machine learning models.
- Declining a legitimate purchase (false decline) because the cardholder is in a different location.
- Blocking a user after an unusual login attempt.
- Denying a refund due to behavior that appears suspicious but isn’t malicious.
False positives also occur due to human error, for instance, during a manual review process. A risk analyst may misinterpret high-risk behavior as fraudulent when it’s actually legitimate.
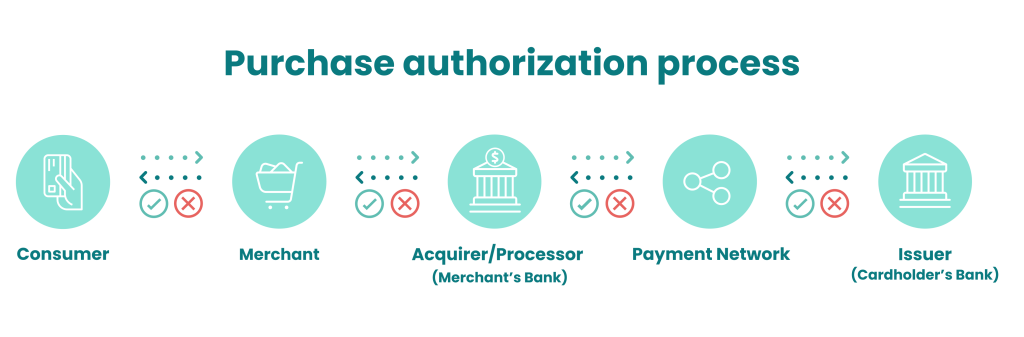
Why Are False Positives a Problem?
False positives don’t just hurt the user experience — they impact your bottom line. Research shows that failed payments are a contributing factor to nearly 50% of all customer churn.
Left unchecked, false positives create a feedback loop. If your fraud system learns from bad data (treating false positives as true fraud) it can become even more aggressive. Leading to more good customers getting blocked and more support tickets piling up.
Are False Positives the Same as False Declines?
Not exactly. False declines are a type of false positive that specifically involves payments. A valid transaction gets flagged as fraud and rejected.
Shoppers dislike both false positives and false decline, and both fall under the “insult rate” umbrella — where your risk engine offends good users by treating them like fraudsters.
5 Ways to Avoid False Positives
Reducing false positives takes a data-driven approach focused on catching fraud efficiently while minimizing friction for legitimate users.
- Audit risk rules: Revisit your risk logic. Are any rules too broad or too strict? Fine-tuning thresholds can make a big difference.
- Test in a sandbox environment: Simulate user journeys in a test environment. If you see high false positives, iterate on your logic before going live.
- Refine manual reviews: Use manual review strategically. Focus on medium-risk events, and compare them with historical data to improve accuracy.
- Leverage machine learning: Use dynamic models that learn from outcomes. A good fraud platform will retrain itself to minimize false flags over time.
- Rethink chargeback guarantee tools: These tools often prioritize avoiding chargebacks at all costs, sometimes blocking good customers in the process. Consider alternatives if they don’t align with your business model.
Strike the right balance between strong protection, sophisticated risk assessment and positive customer experience.
Speak with an expert
Sources