Both SEON and Sift offer solutions for fraud detection and risk management, though they differ in how they structure and deliver key features. The following comparison outlines the practical distinctions in machine learning, rule configuration, data handling, and user visibility.
SEON vs Sift Comparison Table
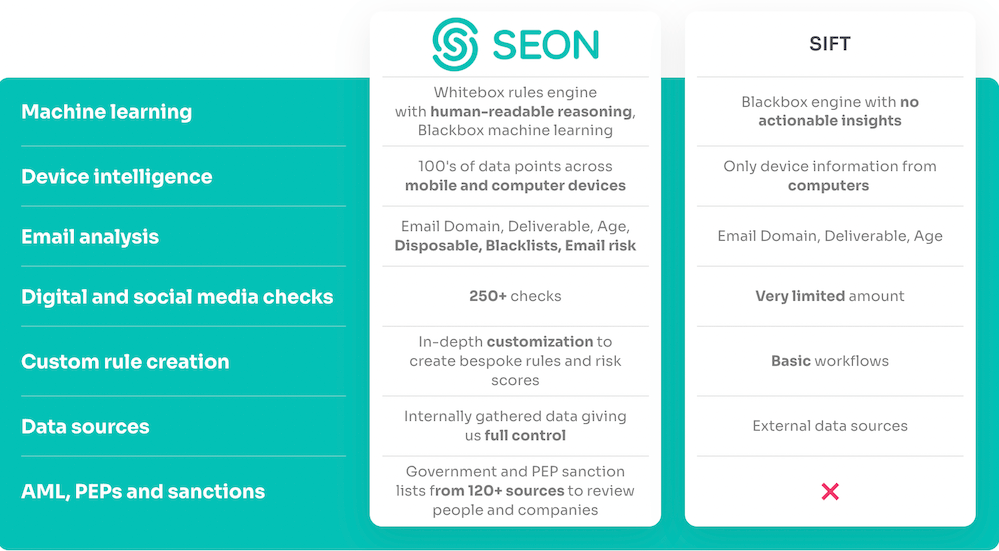
Rule Customization and Control
SEON provides both preset and fully customizable rule creation through a user-friendly interface. This includes support for logic such as data comparisons, pattern velocity, and behavioral signals. The approach allows teams to adapt fraud logic based on internal thresholds, industry shifts, or regulatory changes.
Sift supports custom inputs primarily through API fields. While suitable for teams with dedicated engineering resources, this structure may offer less flexibility for non-technical users managing real-time changes.
Interpretable Machine Learning
SEON’s system includes both whitebox and blackbox machine learning. The whitebox component generates explainable rule suggestions based on observed patterns, while the blackbox model runs in the background to detect more complex, less visible anomalies. This dual structure supports adaptability while preserving transparency.
Sift uses a blackbox machine learning engine to automate risk decisions. This can be effective for scaling fraud detection but offers limited visibility into how decisions are made, which may affect auditability or the ability to fine-tune responses.
Device and Technical Data Coverage
SEON collects extensive device intelligence across mobile and desktop platforms. This includes hundreds of technical data points, helping enrich risk profiles and spot device-level fraud signals that may be overlooked in simpler setups.
Sift also gathers device information but is primarily oriented toward desktop environments. The range of device signals available may vary depending on the integration context.
Use of Digital Footprint Signals
SEON includes real-time checks on more than 200 digital and social signals, offering insight into user legitimacy when traditional credit or identity data is unavailable. Signals such as subscription services, social media presence, and online account activity help provide additional layers of verification.
Sift provides more limited digital and social checks, focusing instead on conventional data signals and behavioral analysis.
Disclaimer: Everything you’ll read in this article was gleaned from online research, including user reviews. We did not have time to manually test every tool. This article was last updated in Q2 2024. Please feel free to contact us to request an update/correction.
Further Reading
Learn more about:
Browser Fingerprinting | Digital Footprinting | Device Fingerprinting | Fraud Detection API | Fraud Detection & Prevention
Try our free tools:
BIN Lookup | IP Lookup | Reverse Email Lookup | Reverse Phone Lookup | Social Media Lookup