For a customer, getting a loan used to be an exercise in red tape and resilience – and that’s if they had the credit to qualify. Now, online lenders deliver loans in minutes, even to underbanked markets. This high-speed, frictionless service is great for a growing loan industry, but it’s also opened the gates to high-risk customers.
Lenders have realized the immense market potential of loans to the underbanked. People who were traditionally seen as unsafe borrowers – such as those who don’t have proof of regular credit card or mortgage repayments – are now being presented with options to increase their personal spending power. The challenge is that without a credit history, they still present an underwriting risk.
Furthermore, lenders hoping to capture the emerging microloan market have to balance frictionless services with the need to block out defaulters. In this article, we help lenders understand how to capture growth while lowering default risk.
Why Are High-Risk Customers a Problem for Online Lending?
There are two types of high-risk loan customers: those without financial security (who are unable to repay loans) and fraudsters (who have no intention of doing so). Regardless of whether a customer represents an underwriting risk or a regulatory one, high-risk borrowers create the same distinct problems for lenders:
- Loss of money from unpaid loans
- Cost of reclaiming unpaid loans
- Resource needed to exclude high-risk customers
- Increased customer friction during customer checks
- Excluding the wrong customers
Fintech provider Solventa cut fraudulent transactions by 25% and boosted the accuracy of its risk modeling by 15% with SEON’s machine learning.
Read case study
How to Detect Borrowers With a High Risk of Default
Digital profiling provides an alternative source of credit information. It uses real-time data to understand whether customers are making regular payments now. This means it can give more accurate insights than historical, often outdated credit data while also being more affordable and attainable.
Using a customer’s phone number, email, or IP address and a digital footprint solution such as SEON’s, lenders can find which online accounts a customer holds. We call these signals. These signals give proof of a customer’s online life, including regular payments and spending habits.
What does the typical borrower’s digital profile look like?
The typical borrower has around 10 online accounts, which each send a digital signal. The most common of which are from:
- Microsoft
A drop of 40% or more represents a risk that the applicant may be a fraudster who is using a fake or stolen ID. We’ll explain more about those risks later in the article.
What does a reliable borrower’s digital profile look like?
Digging further into a borrower’s signals, you can find indicators of financial stability and regular payments. For example, if a borrower has an account with Spotify, they make low monthly payments.
SEON’s digital profiling assesses 90+ digital signals, including those used globally and those unique to certain countries. Our data tells us that the most common signals of a borrower’s financial stability across all regions are:
Apple: A premium product that indicates high income and regular payments
Spotify: Indicates low but regular monthly subscription payments
Amazon: Indicates purchase history and low but regular monthly subscription payments
These are just three of the 90+ digital signals SEON monitors. Finding a signal from one or more of these accounts will lower your customer’s default risk score and give you greater insight into their ability to repay debt.
How to Detect High-Risk Customers in Online Lending
The second type of loan default risk comes from fraudulent customers, who will try to beat your risk assessment by presenting a trustworthy face in one of two ways:
- Synthetic identity fraudsters: Acquire stolen IDs to apply for a loan.
- Phishing scammers: Take over legitimate customer accounts.
To find these high-risk fraudulent customers, lenders should ask:
- Is this person who they say they are?
- Is the ‘person’ real, fictitious, or a mix of both?
- Does this person represent a regulatory risk? If so, what kind and does the nature of their loan application compound this risk?
- Is the recipient of the loan clear, or are they hidden behind complexity?
Risk-based fraud prevention software like SEON does this in multiple ways:
- Device fingerprinting: This detects the unique configuration of a device, catching both synthetic ID fraud and ATO. For example, a common sign of a professional fraudster scaling up their crime is that multiple users with different IDs and locations are connecting from the same machine.
- IP geolocation: This is an important part of detecting the use of synthetic IDs, regulatory risk, and ATOs. New accounts and loan applications with an address that is different from the IP geolocation could indicate a fraudster.
If the IP location is in a high-risk area, fraud teams should dig deeper, and potentially, users should be blocked.
- Geolocation masking: Use of the Tor browser or virtual private networks (VPNs), (and various other proxies) is a common signal of malintent as it allows fraudsters to hide.
- Reverse email lookup: Fraudsters are likely to set up a new email address in their attempt to claim a loan. High-risk signifiers include new email addresses and those that have little history, including data breaches, which most email accounts have some history of.
- Digital profiling: A trustworthy loan applicant will have a digital presence attached to their email, for example, they may have WhatsApp and Telegram. No social media presence suggests a fake account, whereas a trustworthy account will have a wide presence and multiple apps, and these will most likely contain some of the most popular platforms.
Top Three Custom Rules for High-Risk Customers in Online Lending
SEON’s end-to-end fraud prevention solution lets lenders set their own parameters to catch high-risk customers, and our customer service team helps you find the right way for you. Our custom rules can be adapted to match your risk appetite, markets, and customer data.
Here are three custom rules that our lending customers regularly use to filter out high-risk customers.
#1: Multiple Geolocations with Same Device Hash
Loan fraudsters are aware their success depends on convincing lenders that they are who they say they are. A common tactic for a digital loan fraudster is to acquire or create an identity and then create a believable digital footprint that will roughly match that identity. As these false or stolen identities may be from anywhere in the world, many fraudsters may want to develop this footprint by spoofing an IP location near their falsified address or employing a nearby data center.
Device hashes are unique enough that risk rises when multiple users appear to be using the same device. Cookie hashes perform a similar fraud detection function for desktop users. Such instances should be manually reviewed or trigger a higher risk score.
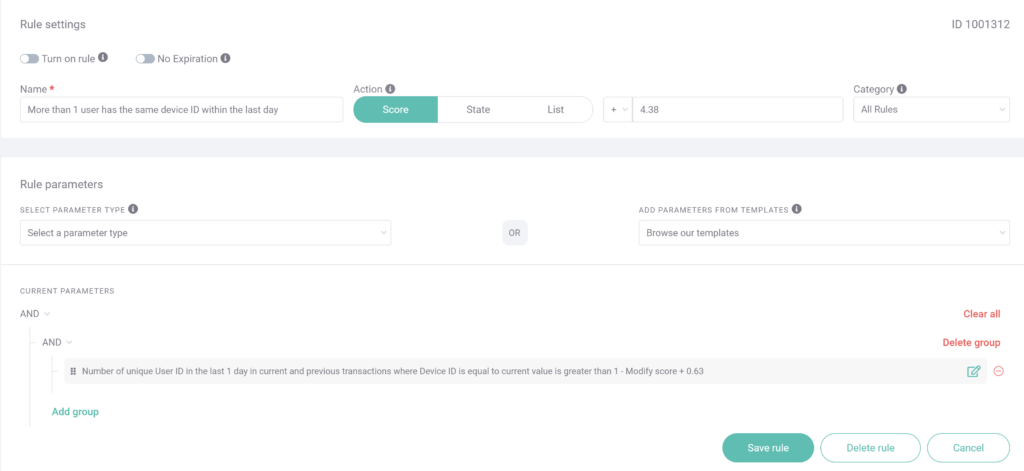
This screengrab from SEON’s custom rule editor shows a rule that has been tailored to this specific case. Here, the rule is scanning for instances where multiple accounts are used on the same device within a day. This is a reliable sign that a fraudster is making several fraudulent loan applications coordinated from one device.
#2: Suspiciously Small Social Media Footprint, Free Phone Numbers
Fraudsters will often attempt to make their fake profiles look normal. This includes adding mobile phone numbers and social media accounts. However, building a realistic social media footprint is time-consuming, especially for fraudsters hoping to scale their crime and maximize illicit profits. It is unlikely that such fraudsters will have the resources to add more than one social media profile, if any.
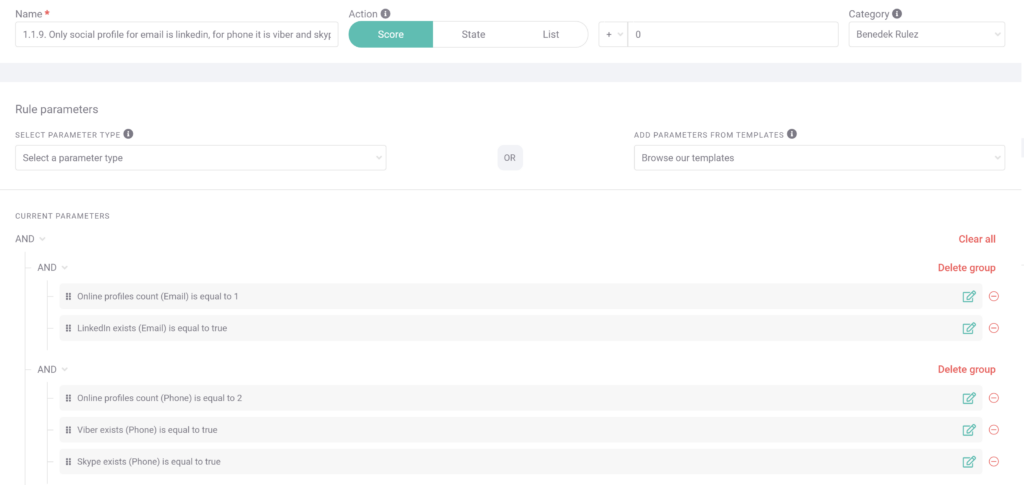
In this screengrab, a custom rule has been set up to detect such anomalies. In this example, a lender is looking for specific social media signals that represent default risk within their customer base.
LinkedIn is a common social media account useful to a lender for signaling employment. However, for this reason, fraudsters are likely to set up false LinkedIn profiles to bypass social media presence checks. However, it’s unlikely that an email account only has a LinkedIn account associated with it, so fraud teams should look for a mix of account signals.
#3: Same User, New Device, New IP
SEON’s custom rules can be used to detect the possibility of an account takeover. Although there may be any number of reasons why an already-onboarded customer might suddenly have a new IP and device hash (such as going on vacation with a new phone), this situation should raise interest in fraud teams with a well-defined risk appetite.
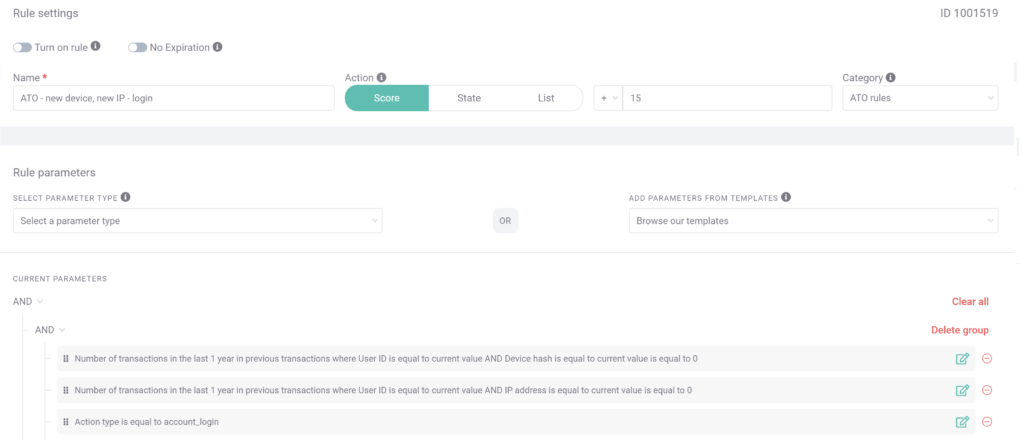
How SEON Helps Online Lending with High-Risk Customers
While online lenders democratize spending power through low-friction loans and BNPL, SEON’s goal is to democratize the fight against fraud. That means arming you with the tools you need not just to detect fraud, but to prevent it from ever happening.
In this article, we’ve discussed only a few of the functions within SEON’s end-to-end fraud prevention solution. Get in touch if you’d like to know more about how to Reduce Fraud Rates by 70–90% using digital profiling, whitebox machine learning, and advanced APIs.
Partner with SEON to reduce fraud rates in your business with real-time data enrichment, machine learning, and advanced APIs.
Ask an Expert
Related Case Studies for Online Lending
- Solventa Leverages SEON’s Machine Learning to Reduce Fraudulent Transactions by 25%
- Revolut Leverages SEON’s Anti-Fraud Platform With Great ROI