What Is Link Analysis?
Link analysis, also known as network visualization, is a visual data analysis technique. It enables analysis of the connections between network nodes, thus facilitating the analysis of relationships within a dataset. This can involve the analysis of links between objects such as transactions, people, organizations, addresses, accounts, and more.
Link analysis can reveal insights that other forms of data analysis do not. As such, businesses are increasingly using it to understand their operations and drive their decision-making.
A wide range of businesses rely on link analysis, as the theory can be applied in multiple ways. It can be used for undertakings as diverse as medical research, security analysis, search engine optimization, and fraud detection.
We’ll mostly be focusing on the use of link analysis in relation to fraud below.
Read how our software stops multi-accounting, loyalty and bonus abuse, account takeovers and more by spotting customer connections.
Read More Here
What Is Link Analysis in Fraud?
In the context of fraud prevention, link analysis spots hidden connections between users of a network or platform in order to catch and potentially block anyone who attempts multi-accounting in order to conduct fraudulent schemes against the company or its legitimate customers.
Link analysis can be a powerful fraud detection tool. Studying the connections between individuals, the devices they use, their shipping addresses, the payment methods they use, and so forth can expose both small and large-scale fraud rings.
This means that businesses that use link analysis are better placed to detect fraudulent activity. With the right strategy, they can even identify potential future fraud.
In practical terms, this can help companies uncover instances of fraud such as:
- multi-accounting
- bonus abuse
- affiliate fraud
- account takeover attacks
- money laundering
- etc.
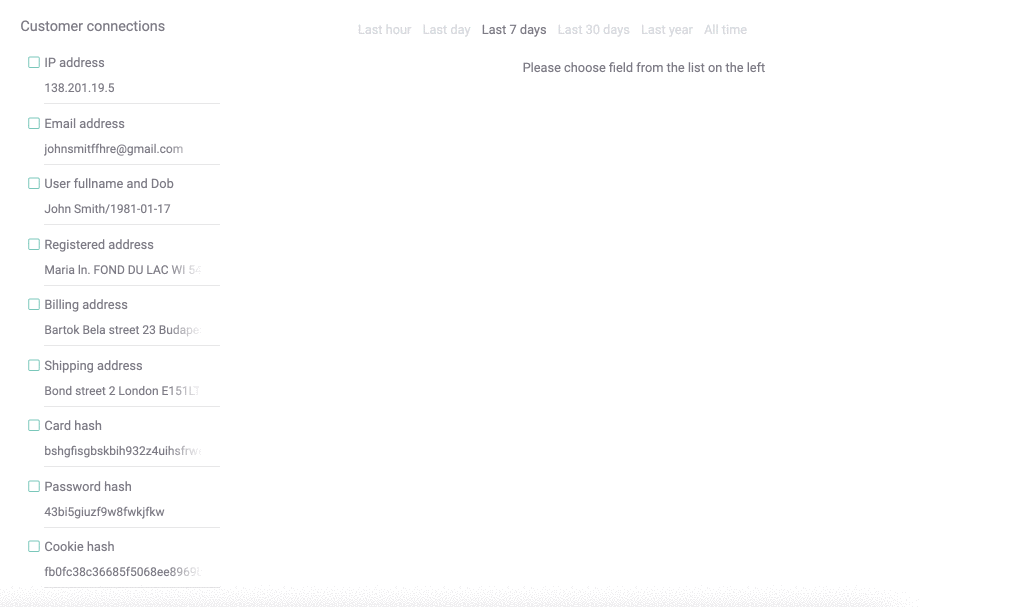
How Does Link Analysis Work?
When it comes to combatting fraud, link analysis works by examining the relationships between all the different nodes in a network. The system will gather data about each user, and create lists and visualizations of how they might be created based on solid evidence – for example, because they have the same browser hash or have been spotted to use the exact same password hash and secret question.
Let’s use insurance fraud as an example. According to the Coalition Against Insurance Fraud, insurance fraud costs American consumers at least $308.6 billion every year. Fraudulent activity related to it spans the healthcare, automobile and real estate industries, along with many other sectors.
Link analysis can help to identify instances of insurance fraud. It can flag up connections between individuals, vehicles and addresses. Unusual relationships between motor vehicle insurance quotes or claims can therefore be flagged up and investigated. This can identify not just fraud that has already taken place but also suspicious activity that could be indicative of attempted fraud.
Another example of how link analysis works can be seen in the online retail sector. A retailer can undertake link analysis to examine the connections between its customers, their payment methods, delivery addresses, email addresses, items ordered, and so on. Any suspicious patterns of activity – such as multiple accounts using the same credit card or shipping to the same address – can be picked up by the link analysis tool and then investigated by the fraud analyst. The results of such detailed analysis can also easily be shared with law enforcement officials.
Banks, too, can use link analysis to identify suspicious connections between account holders’ names, addresses, phone numbers, email addresses, and more, as well as between the accounts themselves and related products. As link analysis can identify connections and patterns that relational table databases can’t, it is enabling banks to identify everything from multi-accounting to synthetic identities.
What Is Link Prediction?
Link prediction is a continually developing graph data science technique that takes link analysis one step further by predicting links that are likely to form, based on those already observed as formed. Like link analysis, the uses of link prediction span many sectors, from medical science to viral marketing.
Link prediction also has various uses when it comes to fighting fraud. It can predict the likely growth of fraud networks, can detect anomalous emails and can predict user accounts that will carry a high fraud risk.
Link prediction is a complex area of data science and there are multiple different approaches that can be taken when using it, including a range of topology-based methods, node attribute-based methods and mixed methods.
The evolution of machine learning models and graph-based models means that link prediction will continue to develop, with further innovations likely as these technologies mature.
Why Is Link Analysis Important?
By understanding customer connections, businesses can catch fraudsters pretending to be someone they are not, and see how many degrees of separation exist between each customer and known or suspected fraudsters. They can then determine which individuals they will and won’t work with, based on their risk appetite.
- This approach can save businesses money by reducing the amount of fraudulent activity that takes place. It can also save them time (which has its own cost attached), as the firms can use link analysis to automate the process of identifying fraudulent and potentially fraudulent activity.
- Firms that use link analysis may also derive reputational benefits from it, again by reducing their likelihood of being defrauded.
- Link analysis is also important for everyday consumers. They are the ones who have to bear the cost of fraud to businesses, through increased premiums and prices that enable firms to swallow the cost of any fraud that takes place. As such, link analysis can save customers money, as well as organizations.
- From a societal standpoint, link analysis is important as well. It can help identify not just individual fraudsters but entire networks of them, and it can be instrumental in putting criminals behind bars and keeping the economy healthier.
- The potential of link analysis to identify future fraud is also important. Machine learning models can help to predict how likely it is that a network could be fraudulent. This can head off instances of fraud before they take place.
Partner with SEON to reduce fraud rates in your business with real-time data enrichment, whitebox machine learning, and advanced APIs.
Ask an Expert
Examples of What Link Analysis Can Uncover
Fraud networks, also called fraud rings, can be found around the world. They regularly span international borders thanks to individual members’ ability to connect via the dark web. They can facilitate the sharing of stolen contact, account and payment card details, and connect fraudsters to one another in myriad other ways.
A recent fraud network example can be found in the boom in synthetic identity fraud. Synthetic identity fraud is where criminals use elements taken from real people’s identities (addresses, social security numbers, and the like) and mix them up to create new, fictitious but convincing identities.
The Federal Reserve has called synthetic identity fraud the fastest-growing financial crime in the US. It cost financial institutions $20 billion in 2020. The average synthetic identity fraudster managed to steal between $81,000 and $97,000 before the fraud was uncovered.
In one of the most high-profile synthetic ID fraud cases, 13 people were charged by the Department of Justice after creating 7,000 fake identities. The fraudsters used the made-up identities to obtain tens of thousands of credit cards, which allowed them to steal at least $200 million.
Telecommunications fraudsters also operate large networks, working together to carry out a range of scams of varying sophistication. These can result in major disruption and significant costs for the businesses and individuals that they target.
An example is international revenue sharing fraud (IRSF) where fraudsters set up premium rate numbers and then call them themselves from hacked business phone systems, pocketing the 25% that they earn from the call costs. Such fraud costs the telecoms industry an estimated $4 to $6.1 billion per year.
The sheer scale of fraud networks and level of collaboration among fraudsters means that those fighting fraud need to use all the tools and methods at their disposal – methods which include link analysis.