Advanced technologies are transforming how fraudsters operate and how companies are fighting back. With AI-generated threats contributing to a proliferation of complex fraud schemes and blurring the lines between what’s real and what’s not, organizations must advance their fraud prevention and detection strategies to overcome the limits of traditional methods – tackling emergent and rapidly shapeshifting threats head-on while ensuring operational resilience in uncertain times.
With its mimetic capabilities, AI-generated fraud is harder to detect and occurs at unprecedented volumes and velocities. Now more than ever, it’s becoming critical to enact accurate and precise detection capabilities to identify and stop fraud as soon as possible, working to keep cleaner, more fraud-free ecosystems that deliver seamless customer experiences.
How AI-Generated Fraud Works: Understanding the Threats
AI-generated fraud represents a significant evolution in malicious activities, leaning on advanced technologies to create and execute sophisticated schemes. One common tactic is fraudsters generating fake documents and data (or using synthetic IDs), all of which are crafted precisely and closely resemble authentic materials. These fraudulent documents, whether invoices, contracts or financial reports, pose considerable challenges to detection, lacking the usual discrepancies that previously hinted at forgeries. As a result, organizations face large volumes of fraudulent data, necessitating heightened vigilance and advanced detection methods to protect themselves – and their customers or users.
Other AI applications include enhancements to traditional scams, such as phishing and social engineering attacks. By personalizing approaches and crafting tailored messages, fraudsters can appear more convincing than ever to unsuspecting targets. Add in the emergence of deepfake technologies that allow fraudsters to impersonate individuals through manipulated videos or audio, adding an extra layer of authenticity to deceitful tactics, and the landscape of fraud becomes even more difficult to parse. Just ask the finance worker who mistakenly paid out $25 million after what he thought was a Zoom call with his CFO or the state of Florida that paid $1.2 million for a purported new headquarters building in an email scam.
Limitations of Traditional Methods & Approaches
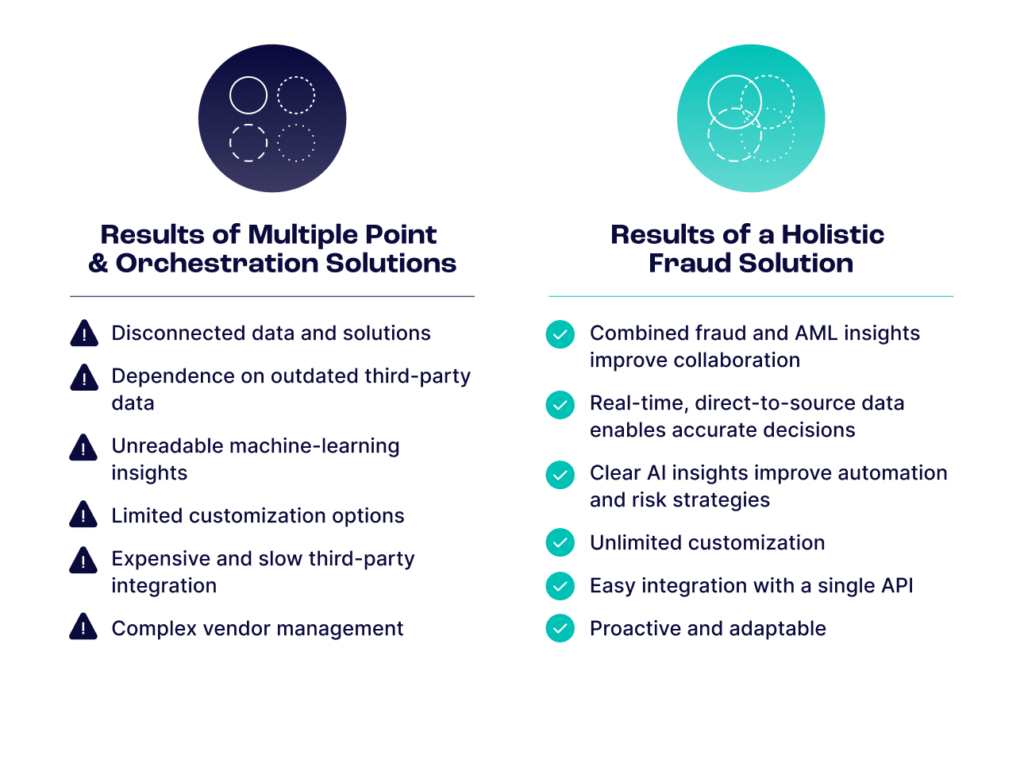
The surge in digital activities underscores the urgency of implementing effective fraud prevention measures. However, traditional anti-fraud methods, such as device checks, two-factor authentication (2FA) and biometric authentication, to name a few, are proving inadequate against new techniques employed by fraudsters. While effective in their time, traditional anti-fraud tools often fall short when confronted with the growing deceptive capabilities available through AI-enhanced threats. Outmoded methods need more scalability to analyze vast amounts of data in real-time or to look at behavioral analytics to digest true user intent, leaving organizations vulnerable to evolving attack vectors.
Historically, companies have taken a fragmented approach to fraud prevention, characterized by a variegated tech stack of point solutions or third-party orchestrators. Today, this approach can generate operational inefficiencies and obfuscate risk views. While the market for end-to-end anti-fraud solutions is expanding, there is a current overreliance on third-party data that disrupts an organization’s ability to access real-time, direct-to-source intelligence or customize solutions to their specific needs – all integral to staying abreast of AI-backed fraud trends tailored across industries.
Further, the traditional standard of fraud-fighting is inherently reactive rather than proactive, meaning the tools respond to known fraud patterns rather than anticipating and identifying emerging threats as they surface. This reactive approach renders organizations in a continuous game of catch-up against fraudsters in the lucrative business of staying one step ahead, innovating tactics to circumvent detection and make off with profits. Without the ability to adapt and evolve alongside changing threats, traditional fraud prevention methods are ineffective in the face of AI-enhanced schemes.
Real-Time Insights through AI
To combat AI-generated fraud, organizations must assume a multifaceted approach integrating the same advanced technologies bad actors use to deploy attacks quickly and scale. AI-powered fraud detection and prevention tools leverage machine learning algorithms to identify suspicious patterns indicative of fraudulent activity and pinpoint irregularities, like unusual transaction amounts or frequencies that deviate from expected norms. Likewise, they can also discern intent by examining behavioral patterns, delivering insight to decipher fraudsters from legitimate customers. Biometric authentication, a technology frequently conflated with behavioral analysis, is inaccurate in identifying patterns and is vulnerable to spoofing. Behavioral analysis empowers AI to track user interactions over time, swiftly flagging activities that diverge from typical behavior patterns, such as sudden shifts in spending habits or transaction types.
There are two types of AI: blackbox ML, which focuses on outcomes without revealing the underlying processes, and whitebox ML, which provides transparent insights into decisions. Understanding and utilizing both methodologies is the best way to serve fraud detection capabilities. Through continuous learning capabilities, AI systems evolve alongside changing threats, ensuring ongoing accuracy and effectiveness in detecting preventive fraud and working to identify new fraudulent activities and patterns once they emerge at the earliest identification point.
SEON’s Unique Take on AI
SEON’s machine learning solution seamlessly integrates the strengths of whitebox and blackbox approaches, complementing the scoring engine that applies default and customized rules to the extensive user data collected throughout the user journey. By simultaneously processing the same user data to identify intricate fraud patterns that may elude human detection, not captured by the default and custom ruleset, the blackbox component generates a fraud risk probability score to evaluate risk. In contrast, the whitebox component suggests new risk rules to implement. Once approved, these suggestions enhance the custom ruleset. Both components continuously retrain themselves with new data, resulting in increasingly precise risk probability scores, refined rule suggestions, and, ultimately, more accurate automated risk decisions.
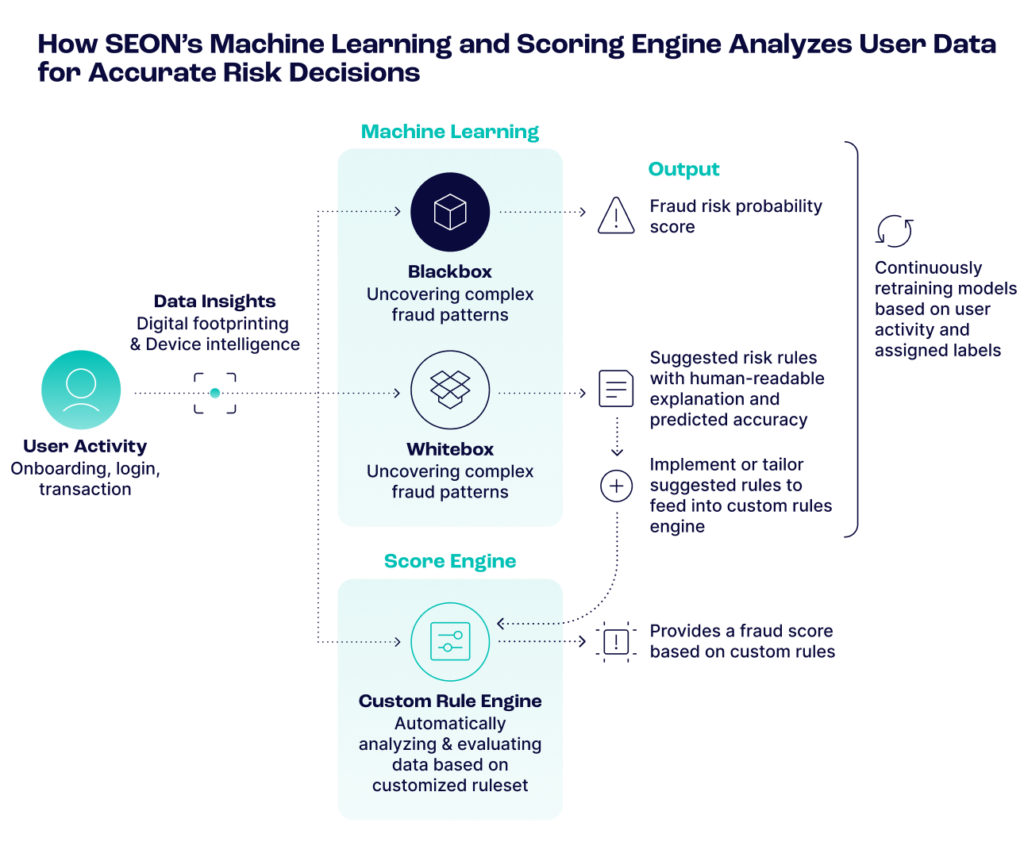
Strategic Advantages for a Resilient Future
As AI continues to reshape fraud’s call and response, using advanced AI for detection and prevention is not just an option but a necessity. By navigating practical challenges such as data privacy, model interpretability, integration hurdles, and the need for continuous monitoring, businesses can forge resilient systems capable of defending against evolving fraud tactics. These actions enable enterprises to build dynamic, adaptive and agile capabilities essential for combating fraud in its current iterations and whatever comes next.