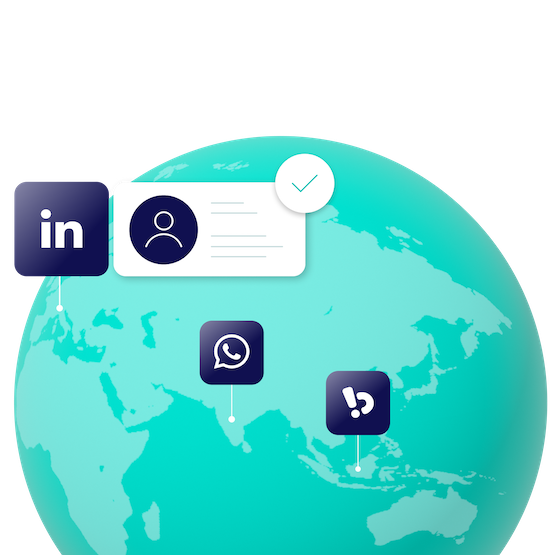
How to Assess Credit Worthiness Using Alternative Data
In this webinar you’ll learn how digital profiling can reveal your customer’s income and credit risk, helping you expand globally while reducing your risk of loan default. We’ll present our global data to share the regional insights and trends we’re seeing.
We’re thrilled to be joined by Cecilia Lopez, Head of Decisioning at Carbon, a leading loan app in Nigeria. She’ll be sharing her own experiences and best practices using digital profiling to assess credit risk.
You’ll also learn:
- How alternative credit data solves some of the shortcomings of traditional credit scoring
- The typical digital footprint we see across regions
- Digital signals that can indicate wealth and disposable income
- Best practices, insights and lessons from a peer in the industry
Guest speaker:
Cecilia López
Head of Decisioning
Carbon
Cecilia López leads the Data Science and Credit Risk teams at Carbon as the Head of Decisioning. She is an Actuary with 15 years of experience in banking, predictive modeling, and risk assessment, specializing in credit risk, as well as the optimization and automation of business processes.
Date/time:
Thursday December 7, 2023
10AM EST / 4PM CET / 8:30PM IST
Webinar Transcript
INTRODUCTION
– Hello and welcome to our webinar on assessing creditworthiness using alternative data. In this webinar, we are going to cover global and regional insights and we are going to specifically look at new methods using real-time digital data in order to best assess your users and your customers’ creditworthiness and their risk profile.
With me today is Cecilia Lopez from Carbon, who is Head of Decisioning. Cecilia, thanks for joining us. Would you like to introduce yourself in a few short sentences?
– Sure, hi Daniel, thank you for having me. I’m Cecilia Lopez, I’m the Head of Decisioning at Carbon. I’m an actuary, I graduated from Buenos Aires University. I’ve been working in the financial sector for almost 15 years now, particularly in banking, credit risk management, and predictive modeling.
CHALLENGES WITH TRADITIONAL DATA
– Thank you. What we want to dig into today is how, globally, people can use alternative ways to assess their customers’ loanworthiness and creditworthiness. A good point to start at is just looking at some global statistics. We’ve looked into this and in the world, there are about 1.4 billion unbanked and underbanked adults. And that is an absolutely staggering number, right? And it’s staggering for multiple reasons.
But again, chief amongst which is the fact that this makes credit decisioning incredibly difficult. We’ve seen multiple shifts in the last few years in terms of how companies utilize data and find creative ways around that challenge. I would love to hear your thoughts on that. How do you see that trend shift, and how have you seen it shift in the past few years, and how do you see it shift in the next few?
– Absolutely, yes. So, working with just traditional data in the past has been challenging for us at Carbon as well, and for most digital banking institutions. And while this data, this traditional data we used in the past, while valuable, often fails to capture the evolving financial behaviors of individuals, especially in today’s economic landscape which is so dynamic. And these limitations, of course, led to instances where, in general, the decision inflows, and in particular for us at Cardbon, our loan decision inflow may not fully reflect the financial reality of our customers, of our whole customer base, and that impacts the accuracy and the precision of our risk assessments.
Consider a very simple scenario: a customer’s financial stability and behavior can change rapidly in the face of significant life events. The customer is getting married, and starting a family, and these pivotal moments have profound effects on the individual’s financial standing. This challenges the reliability of historical credit data and the traditional data that we used in the past for risk management. In our case, particularly for Carbon, we operate in Nigeria. Nigeria presents its own set of challenges concerning the access to credit data. And the traditional credit data is often scarce as well.
You need to explore alternative data sources; it’s imperative. This influences our ability to make predictions to assess the creditworthiness of our customer base. We need to integrate new data sources, alternative data, and social data, like the one that is provided by SEON, into our existing processes.
DIGITAL PROFILING
– Absolutely. I think you hit a very important point, which is that oftentimes, the data you have available to you, particularly traditional credit data, might not actually be very descriptive of someone’s current standing. And that’s the challenge, right? On top of that, you said your team needed to adapt constantly. They need to find new data sources, they need to find reliable data sources, and they need to incorporate that because, ultimately, what you’re trying to do is do right by the customer. You never want to decline a loan application from a legitimate user for the wrong reason, right? That is basically stacking one bad situation on top of the other for the end-user.
Let me take a quick pause here to explain what we mean by digital profiling and social signals. Traditionally, companies, loan companies, Buy Now, Pay Later companies, neobanks would look at traditional credit history, your history in your bank account, and your credit history. That is what companies would default to. But nowadays, there are a lot of people without any of those traditional data points. And even if you do have that, the question arises: Is your historical behavior truly indicative of your future behavior? When we speak of digital signals or social signals, what we mean is what we can see by looking at, for example, your presence on specific social sites and your usage of subscription services, which we can see when we look at your email address and your phone number. We’ve seen a tremendous shift in companies realizing the need for this type of alternative data and them incorporating these signals with great effect into their workflows, their day-to-day operations, and crafting a better overall profile for their end user.
HOLISTIC APPROACH
– This also highlights the need for a more holistic approach to credit management and risk assessment in the whole risk framework. We need to embrace the incorporation of alternative data, and not as a replacement for traditional data, but as a complement so we can see and analyze the creditworthiness of our customer base from a more holistic approach. This is a benefit not for our lending portfolio or for the lending portfolio of any banking institution, for the stability and the health of the portfolio, but also for the customer. We get to know our customers better. And when we do that, we can make better lending offers.
WHAT SEON CAN REVEAL ABOUT YOUR CUSTOMER
– Looking at an example of what you see through the email address and the phone number, in this particular instance, we are checking a made-up person, John Kelly. Off of the email address, we can immediately see that he is a senior graphic designer, lives in Las Vegas, and has an Airbnb account with a creation date and a review account date. There is quite a lot you can infer from this: for example, the fact that the job title aligns with the sites he’s on, for example, Adobe, makes perfect sense. This should indicate that this person is probably a safer bet to approve. Likewise, there are a number of subscription services, including Disney Plus. You get this immediate idea of how trustworthy John is, how likely it is that John is a real person with a real wallet and real affordability, and you can make a fairly confident decision in terms of how you want to treat this user.
IMPACT OF TRADITIONAL CREDIT DATA ON WORKLOAD
– What does the workload look like for you and your team on the day-to-day? I imagine having to have that level of agility and adaptability has got to be a challenge.
– Absolutely, you need to react quickly. You see fraud trends, see the pattern, you need to react, and you minimize the impact on the portfolio. All these challenges we were discussing about traditional data had a substantial impact on the team and their workload. Also, the tools we were using to process to explore this data that was also an important point because of the amount of effort, the manual efforts we needed to process the data, explore it, discover insights, to model the data, especially when the data was not readily available, demanded significant resources.
And not only from data science, which is what everyone expects: it was a huge workload for credit risk and for engineering as well because we needed this coordination between all teams to create the rules, do feature engineering, do hypothesis generation, and pass on the rules to the developers at the end of the day to implement those in the tool we had at that moment.
This was a very labor-intensive process. I can give you an example of implementing a rule: it took 3 to 14 days before integrating SEON, which is a lot, and I’m talking about implementation only, just putting the rule in the system so we could use it. I’m not counting here the time of actually discovering the pattern and designing the rule, and thinking of the thresholds and how we could integrate it into the framework.
The change we see from that point in time before we integrated SEON to now, where we can configure a rule in 2 minutes, is very, very impressive. Also, we now have the ability to react quickly. As I was saying, you need to react quickly. When we start seeing changes in the triggering rates of the rules we have implemented in SEON, we can react. We can create new rules, we can change the thresholds, and we don’t have this lag we had before, like two months between rule creation and implementation. And then, fraud is over and it’s in your portfolio. Right now, when we monitor this daily, we see the changes in the patterns in the trigger rates. We have a meeting with the team to understand what needs to be done. We configure the rule in 1-2 minutes, we test it, and it’s there in production. And we can stop the fraudulent attempts that we were seeing almost immediately.
And it was not only about credit risk: this incredible workload we had in terms of putting together the fraud rules also affected other processes. We needed to have a more stringent KYC process back then for meticulous data analysis. The impact was not only on the teams configuring the rules and engineering, and implementing the rules in the system, but also it was about the customer experience. KYC was longer, and the time it took for us to analyze a loan application was longer, so the whole customer experience was worse.
– That is absolutely fascinating. And I’ve got to tell you, it’s flattering to hear that SEON has had that impact on you. It sounds like your challenges were really in two different buckets: one challenge is finding the right data and potentially moving away from outdated, more traditional data sources to more live data sources that are snappier, more descriptive of someone’s current status in the here and now rather than potentially two months ago.
But then the other challenge is decisioning on that. Which, as you said, is a cross-departmental challenge and a cross-departmental opportunity because, frankly speaking, if you don’t have your credit and risk department if you don’t have your engineering, and then your data science teams come together, no one team can do all of that heavy lifting on their own.
CARBON’S APPROACH TO CREATING RULES WITH SEON
– You’re tackling these two challenges to find the right data and essentially to create a system where you can then act on it and decision on it. What’s your general approach? What’s the general rule of thumb at Carbon? First, find the right data, and then create a system where you can truly be adaptable and agile in how you incorporate it?
– Today, that is much simpler, as I was saying. We have the data available. We can check the SEON dashboard and see how the rules we implemented are triggered. We have a core set of 150 rules, and that evolved with time to more than 200. And we are constantly monitoring the triggering rates.
That information is readily available for us right on the dashboard. We can see the triggering rates, and we can see if there are any peaks for a certain rule or any peaks on acceptance or declination rates. We have the data in our databases, and we get the team to check it. We try to understand the velocity of the changes in the patterns.
Then, we get together, we design the rule in terms of the data we have available in SEON, which is again related to the rules that we’re triggering a lot. Then we go from there, we configure the rule, and we put a threshold that is, of course, linked to our risk appetite. We keep the rule, depending on the urgency. If it’s something for which we have time to test more extensively, we may have the rule in there with no impact in the decisioning for one day, a couple of days, maybe if the change is big enough, seven days. After we’ve analyzed the data on how this new rule triggers, we move it to production, which is as simple as changing the score. We change the score from zero to X, whatever matches our risk appetite, and that’s it.
MOST IMPORTANT DATA POINTS USED
– It’s a fascinating process how you’ve gone from this taking two weeks to now potentially taking less than half a day.
I had a conversation with someone in a similar role to yours where we were discussing the trends they’ve seen in terms of which data point has really become most impactful in recent years. What that person said to me is it’s a bit like picking your favorite child. Ultimately, you need all of them, and you want to have all of them. But I think it’s also fascinating. Some people swear by traditional credit bureau data. Some people swear by IDV. Some people think device fingerprinting and IP is the be-all and end-all. Some people think it’s in the email and phone. Have you seen certain patterns emerge and some data points becoming more relevant and more dominant in the last couple of years?
– We use all of them. As I mentioned, we have a big set of rules, over 200, and it’s continuously evolving. We use the data fetched from emails, phones, IP addresses, and the data we collect through the SDK. But in particular, email and phone-related data points are very useful for us.
We are able to detect patterns when we can provide these data points, and we are able to then use all the data that was fetched around them in more than 70% of our rules. In some cases, it’s not the main data point of the rule, but if the rule is based on something else, it might be some data that we also collect, and we also feed the platform with data we collect on our own, so we can combine that data with the data points that we get from SEON. I was saying that even in the cases where the main data point of the rule is related to a data point we collected on our platform, the data we get from emails and phone numbers is a complement in more than 70% of our rules. I would say those are the two more important data points.
And there’s a third one, which is also important, is related to the physical device from which the connection is being done. There are insights from which we can tell with a good level of accuracy if we are in the presence of some coordinated fraud attacks.
ADAPTING HIRING AND TEAM SKILLSET
– That’s fascinating, especially the over 70% correlation between external data to your own data from email and phone. I’m curious, because you’ve already talked about some of the changes that happened in your team and your structure as you revamped some of your processes: I imagine even just hiring someone who has never worked with traditional data, you could easily hire someone with a decade of credit experience. But simultaneously, that person might have zero years of experience working with alternative data. So, how do you overcome that challenge? You still have to grow your team, even though you can automate more today. How do you continuously transform your team so that they are on top of all the new data points that they have to work with?
– Actually, with this process where we changed the system we were using, one of them being SEON, of course, we had a change in the skillset of the team. Before, it was quite intensive in data science and traditional data science methods, being able to dig the data to run certain algorithms or modeling algorithms to create machine learning models.
It was quite intensive in that sense. After we integrated the platform, we were able to migrate to a different skillset where we have very valuable team members who have a comprehensive view of credit risk but are not data specialists. They still have an understanding of what credit risk is and the factors that affect the risk level of a customer.
These team members are able to onboard fairly quickly and configure the rules themselves, and test them using the data that we are collecting through the platform in combination with the data we are collecting through our Carbon app. The whole skillset of it, or the distribution of the skillsets of the team has changed. We still have a data science team, of course, and traditional credit risk functions, but we also have people who join us with a different background, maybe more specialized in credit risk.
Also, the nature of the social data collected through SEON is something quite easy to understand and friendly and something we deal with in our lives, all of us, right? We all use social networks, most of us have a smartphone. So it is something pretty straightforward and friendly. These new team members who have risk expertise that is not necessarily with a focus on data science are able to use the platform almost immediately and to create rules that impact production in a couple of days.
– That’s fascinating, and it’s good to hear that there was this organic shift in terms of skill sets within your team.
TOP DIGITAL AND SOCIAL SIGNALS BY REGION
– Let me show you this slide we have on the top digital and social signals by region. The very first thing we can see here is that the lower the volume of social signals you have, the higher the risk you probably are. I think that makes sense because, looking at all the regions, you would expect your average user to have anywhere between 8 and 11 different sites tied to their various data points, including the email address and the phone number. Then, in addition to that, what we can also infer from these is how much disposable income they have and what is the potential affordability when looking at this end user.
I wanted to run something by you: this is the distribution of specific social signals that we’ve seen in specific regions globally. Carbon operates in Africa. And to me, Africa is actually the standout here for one reason and one reason only, which is that, when you look over almost every other region, you will have apps like Spotify, and you will have providers like Apple at the top. All of those indicate a level of spend: Spotify is a subscription service, and in order for you to have a large concentration of Apple accounts, a lot of people have to have Apple devices. Africa is basically the only place on Earth where Twitter, Instagram, and Pinterest are the most dominant in the top three, all of which are entirely free platforms, with Spotify, for example, being in the middle of the pack. Has any of this surprised you when you started digging into data?
– Actually, yes. I’m also from Latin America, as you know, and there are some patterns that are quite similar. So, I wouldn’t say surprised because I’ve seen some patterns we see in Africa and in Nigeria particularly repeat for Latin America, even if it’s not exactly the same here. We leverage this data in our risk framework.
– That’s awesome. Another slide we had was on signals that indicate potential affordability. And I think there’s quite a lot, right? I mean, I’ve already mentioned Spotify and Apple, but everything from Airbnb and the number of trips you might have taken to LinkedIn and your job role, all the way to other subscription services like Disney Plus or Netflix. Again, have you seen specific patterns emerge in recent memory, and how do you expect this to change in the next few years?
Because as an end-user, what I’m seeing is that there’s a new subscription service popping out almost weekly at this point. Frankly speaking, I’m very confused when I have to pay my subscriptions monthly because, in a few years, it’s basically gone from the top two to now having to keep track of a dozen. I’m curious if you have any expectations about this data continuing to be dominant and become even more popular and how all of this is going to tie into your day-to-day work, where you have to use this data to make better decisions.
– Absolutely, we use this data today in our rules. We don’t use it alone; we use it in combination with other data sources because the fraud techniques to get inside systems become more and more sophisticated with time, so we need to make sure that we don’t rely on a unique data point or subscription signal to run it right. Again, we use the data point in combination with many other data points.
We are seeing this trend you are mentioning: people have more and more subscriptions. I experienced that myself. Like you, I had only one, two, now I have 10. And renewing every year or monthly. But yes, this data becomes important, and also, there is a correlation between this data and some kind of creditworthiness assessment in some cases or KYC process that is done by someone else. When you get this subscription, you are also sharing data with the company to which you are subscribing, and they are doing their own KYC. So this is an additional check that you are able to leverage if you have access to subscription data on your end.
ALTERNATIVE DATA TO SCALE INTO NEW SEGMENTS/PRODUCTS
– I think that’s really interesting you mentioning that this is also making its way into how other departments function, and I imagine these are also products within Carbon. So I’m curious: has using alternative data or social data helped you break into and scale within new segments?
– Actually, yes. Not really a population segment but a new product. When we integrated to SEON, we started with consumer lending or standard consumer loans. We used the platform for a few months for that product only. Then, when we started seeing that we were more efficient and we were able to automate the whole fraud process with the tool, we integrated it into our other product, which is buy now pay later, our BNPL product. And now we are using it for both.
The good part of it is that we didn’t need to build another framework for this. We added one extra data point to the request payload we were sending to SEON, and we distinguished the products and where the application was coming from, if it was from consumer lending, or if it was from the BNPL product. That data point then became available in the platform for us to configure the rules. So we just pick the rules we believe can applied to this new product and use it.
We could also use our core set of rules, like this set of over 150 rules that are the core part of our fraud framework; we are reusing these rules for BNPL. The integration to the new product was quite straightforward, and as we didn’t have to create, design, to implement a whole new risk framework or fraud framework for the new product, we were able to launch fairly quickly.
FIRST TIME DEFAULTS VS. DEFAULTS THROUGHOUT THE JOURNEY
– That’s awesome. From my conversations with people in similar roles to yours and with various neobanks, BNPL providers, and loan companies, I’ve heard that most companies face two challenges: when it comes to credits and defaults, they usually bucket their challenges into two large groups. One is the first-time payment default, which is considered to be the worst of any type, and then all subsequent defaults that may happen halfway through the journey or the repayment process. So, as your team has adopted new data, new practices, and new processes, have you seen a market improvement in preventing first payment defaults, or is it more throughout the journey where you see the improvements?
– Actually, it’s both. I would say there are three aspects to this. The first one is that with the tool, as we are tackling fraud, we are tackling the first payment default directly, which then becomes all payments default, as it’s fraud. So, we put the fraud checks at the beginning of the process, and that, of course, impacts the defaults because you are taking out the fraud component at that point, but also taking out the fraud component at an earlier stage leaves us with a more clean flow afterwards.
We retrained our machine learning models, the ones that our data science team trains to assess creditworthiness. Then, we were able to make the target for those models more specific because we removed the fraud component at the beginning. Now we are focused on these other defaults, the ones you were mentioning, that often happen later in the history of the loan. When we made the target more specific, we were able to achieve higher levels of accuracy in the model. We had a reduction in loan defaults at the beginning because of removing the fraud component. We have a reduction of defaults because we removed the fraud component from the target, so the model performed better.
The third component is that as we are able to react quickly when we see changes in the trends or in the patterns, we can stop fraud immediately. So, if there’s something that was not considered in our set of rules, but we are seeing a strange pattern, we are able to put together a rule quickly. We can stop fraud almost immediately and we prevent those loan defaults at the end. I would say it’s in these three aspects that we saw an improvement in the default. It’s not only about FPD and fraud itself, it’s because we are able to react quickly and because the performance of our models is better because they are more specific now.
– That sounds quite incredible and somewhat surprising to me. Now that you explained it, it makes perfect sense to me that if you remove the obviously high risk from the beginning of the funnel, then you basically give your model more opportunities to refine itself around potentially legitimate people, but people who aren’t necessarily in a good place to repay the loan instead of just catching the obviously bad ones.
UNEXPECTED ADVANTAGES
– I’m curious whether beginning to work with alternative data, particularly social data, had any sort of unexpected advantages where you didn’t expect it to have an impact, but now, having worked with it for a while, you see that it helped?
– Yes, I’m not sure if it’s a surprise, but we didn’t expect the impact to be so big. When we incorporated this data into the underwriting process, we knew that if the data was predictive, it would be effective, and we would be able to reduce the loan default. What we didn’t expect was this ability to actually react so quickly in terms of automating the reactions.
We see a change in the triggering patterns, we see a change in the data points, in the distribution of the variables. We create a new rule and we put it together. Also, we have these velocity rules available that automatically check for repeated instances of behavior. We are able to increase the fraud score as we see an increase in these behaviors automatically. Sometimes, we don’t even need to sit down and analyze the pattern and agree on a new rule and implement it. We just configure a rule that will increase the fraud score of the customer if the pattern repeats for that customer and other customers who are applying at the same time.
That was actually quite impressive for us because we were not only in a better position by being able to configure a rule in a few minutes when we saw a change in the patterns, but we were also able to configure rules, just in case, with the velocity pattern in the rule, so it was automated.
DATA SCIENCE AND MODELLING APPROACH, BEING DATA-DRIVEN
– Adaptability is also very essential. You’re essentially projecting and predicting a potential risk, and you can set up a system, and even if it never happens, you just have an extra rule, and it never triggers, and it’s fine. But if it does, you already have your first line of defense in there.
Which I think is a good segue. One of the challenges that I keep hearing from many industries is that one, it’s good to work with new data, with new types of data, but two, that it is inherently challenging. If you’ve never modeled with it, if you’ve never worked with it, you don’t have a blueprint. You can’t just download the recipe like hey, here is how you incorporate that data; here is how you get value out of it. For many companies and for many teams, that’s an ongoing challenge to figure out what to do with this
From the sound of it, you and your team figured this out quite quickly. So, what was the secret sauce? How do you go about just deciphering new data and making sure that there is always value you get out of it?
– There are two things. We still work with the data science and modeling approach where you create the hypothesis, and you’re thinking about how that hypothesis or that new feature you’re targeting with the hypothesis is correlated to fraud. There’s a lot of intuition in there, a lot of expertise. We have an amazing team with years of experience in credit risk, but we keep this approach. We create the hypothesis, we make the statement, we believe this data point should be positively or negatively correlated to fraud.
But we also check the data, of course, at the end of the day. We normally collect data for a few days so we can then take a look at it and confirm our hypothesis. Then, if it is confirmed, we just create the rule and put it in production, but it’s a combination of intuition and expertise, not only in the industry, in credit risk, but also as users of social networks, for example. Our whole team is data-driven.
This change in the skill sets of our team is about that: data is not only a thing of data science or business intelligence at Carbon anymore. Everyone works with data; almost everyone has the ability to get into the database and make the necessary checks to confirm their hypothesis or their proposals. So it’s a combination of these three things.
CREATING A NEW FRAMEWORK FROM SCRATCH
– I appreciate the detailed answer. I think this is a good segue because I realize I asked you quite a lot of questions, and I asked them through my own lens of what I think is interesting and tied to this topic. But I’m curious, what is the question I didn’t ask but you think is absolutely fascinating? Any sort of anecdotal stories, maybe from your journey at Carbon or anything that you find is an underrated thing to ask about and to talk about within this space?
– I would say that could be about integration. When you move from the system you built in-house, totally customized to your situation, to your risk framework, to your risk appetite, it’s hard to move to another world where you use a completely different tool and need to start from scratch. You need to create a whole new framework because the one we had, which was great before, is not flexible enough anymore.
So, where do we start? In our case, we started by trying to replicate the rules we had in our framework, which, again, were about 10 or 12, not a lot compared to the 200 we have now. We started there, and then we tried to leverage all the extra alternative data we got from the platform that we didn’t have before. We work with SEON’s team to configure the rules and train our staff on how to configure the rules. We also relied on rules that SEON has pre-configured. In some cases, those rules apply to our use case. Some cases don’t, but we took these initial set of default rules and used them as they were at the beginning so we could have a quick start.
Then, we migrated these default rules to something more customized, changing the threshold, changing the scores, and adding and combining them with data points that we are collecting on our end to make it more customized. We were able to move from an in-house system to these other products, starting with a replication of what we had in a much more friendly way and the standard set of rules that SEON provided. And then, we migrated to where we are right now, which is a completely customized fraud framework taylored to what we need.
– Spot on! Integration is probably the right answer to what’s the most underrated, not talked-about thing because it’s one of those things where if it goes wrong, everybody talks about it, and everybody tries to forget it really quickly, and if it goes right, you just go like, okay, nevermind, it worked, let’s move on now.
OUTRO
– Cecilia, I think it has been absolutely fascinating to get your insights and some of the behind-the-scenes thoughts, and just the general logic and approach of how you solve a real problem. It’s not just about credit defaults or fraud: it’s about how you roll with the punches, so to speak, how you stay on top of your business, which, I think, Carbon clearly does.
Before I let you go and before we say goodbye, what’s next for Carbon? What’s the next big thing? I know you obviously can’t reveal everything, but what do we have to look forward to in 2024?
– A lot, but I say we are now putting the focus on our customer experience. It’ll be all about customer experience and the use of tools like SEON to help us towards that, meeting that objective. If we want to have a great customer experience, we need a tool that is easy to use, and processes that are straightforward, where most of the analytics and decisioning is happening in the backend with the user not even noticing. It’ll be all about that. Trying to improve our processes even more to provide a great customer experience for our customer base. We’ve served over 1.8 million customers so far, and we expect to grow a lot in 2024. This is our focus.
– Definitely looking forward to seeing more of that. I’m quite curious to try out your app now. Well, Cecilia, thanks so much for joining, and thank you for sharing your insights. Thanks to everyone who has watched us and listened to us. If you have any questions, please feel free to reach out to us. Thanks, everyone.
– Thank you, Daniel, it was great. Thanks a lot.
Speak with a fraud fighter.
Click here
SEON Resources
Sign up for our newsletter
The top stories of the month delivered straight to your inbox