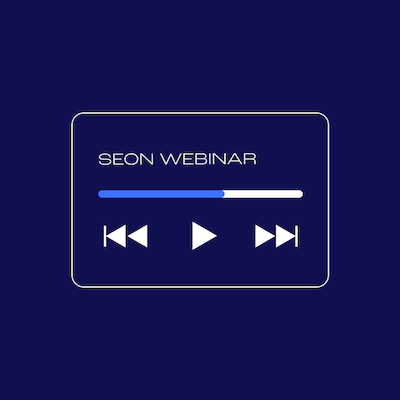
AI in Payments: Balancing Security with User Experience
This Wired-In webinar hosted by NorthRow welcomed SEON CEO Tamas Kadar to explore the pivotal role of artificial intelligence (AI) in the evolution of payment systems.
As digitalization accelerates, AI’s integration into payments enhances security and customer satisfaction. The session sheds light on balancing rigorous security measures and maintaining a seamless user experience and will also address the main fraud challenges, the shortcomings of traditional fraud prevention, and how AI can fortify payment systems without compromising customer experience.
This session delves into how SEON leans on AI and machine learning to stop fraud before it happens. You’ll learn:
- Why we need to lean on AI and machine learning for faster and more accurate fraud prevention, and how you actually do it in practice
- How AI also supports seamless payment experiences, driving customer satisfaction, trust and loyalty
- How AI is shaping the future landscape of payment fraud prevention and drives SEON’s innovations
Watch the replay for insights into the future of fraud prevention and the transformative impact of AI in the payments sector.
Webinar transcript
Reece: Hello everyone. And welcome to the latest installment of Wired-In. I’m your host today, Reece, digital marketing lead at NorthRow. A big thanks to everyone who has joined today. The session is going to be hosted alongside the fraud fighters of SEON as we welcome their founder and CEO, Tamas Kadar. We’ll be addressing the topic of AI in payments, which we’ll touch on more in a second.
Before we jump in on a bit more about Tamas and a bit more about the session at hand, I’d like to bring some awareness to who NorthRow are and what we do. So Tamas, if you don’t mind putting the slide forward, please. Awesome. So essentially, NorthRow provides a compliance platform that covers KYC, KYB, and IDMV and essentially just helps you to make decisions faster, onboard clients in seconds, and comply with the ever-changing legislations.
So, if those issues resonate with you, head over to our website and let’s go from there. Just a few housekeeping elements for today’s session. You’ll see it on the panel to your right-hand side, and you can move this about wherever you like to fit on the screen. For audio, you can switch between dialing from your phone and dialing from your laptop or device. If you have any issues, we will have an attendee on the line here to help. Use the comment section for the questions; please submit any in the Q&A panel as well. We forward these to the SEON team, and Tamas can get in touch later on. The session is also being recorded, so it’ll be live on the NorthRow events website. It’ll also be live with SEON, but we’ll also send an email copy of the recording. So, Tamas, just one more for me, please. Perfect. So a bit about our special guest today. So Tamas Kadar is the founder and CEO of SEON. He started the company with his co-founder when they were still students in university and built it from scratch.
A graduate of Corvinus University, he saw firsthand how fraudsters and hackers look to get around security measures. He’s featured in the Forbes Hottest Young Startups in Europe and is a regular startup pitch winner. He’s a true tech enthusiast and product visionary for creating a fraud-free world and has recently been included on the elite 30 under 30 Forbes Europe list as the face of the technology list. So, without further ado, the floor is yours.
Tamas: Thank you so much for having me on this webinar, a pleasure to be here. What I would like to begin with is just an overview of the main fraud challenges businesses can face in the payment sector today; it can range from customers using stolen cards, which can lead to chargeback fraud, as well as stolen passports, which can lead to account takeover attacks.
We’re seeing an increased volume of money mule scams when they’re using stolen or synthetic identities to create dropback accounts. This can lead to money laundering, which is another issue and this is part of the broader landscape of anti-money laundering projects.
The last two points would be card testing, which is, since the very beginning, a very frequent form or process to try and see if the card numbers they have access to are valid or not. They can use multiple ways of charging the cards for small amounts and see whether they are live or not.
And, of course, businesses need to be compliant with the regulatory bodies. Following the traces of incoming and outgoing money flows, looking at sanction lists, crime lists, and the velocity of money movements are all part of the regulatory scheme that has been rolled out over the last 10 to 15 years.
And with the increase of digital banks, this is at the forefront of the new challenges. Moving on, what I would like to show here is how the new upcoming four-dimensional solutions have dealt with the new form of digital payments. So, in the very early days, most of these traditional fraud prevention solutions were mainly focused on this transaction phase.
This was coming after the normal onboarding/KYC procedure. Also, it’s part of the broader customer due diligence initiative. What SEON has built and what I think every online business should take care of is tracking the customer from the first, earliest access point in their system. This starts from onboarding registration, but even a step before, when a customer lands on your website or app, when you have to begin your investigation. And then every action, every step of the customer’s journey, are very important part of the behavior. The more data as an online business you have, the better decisions you can make.
Fraud prevention solutions, like what we have built at SEON, are designed to stop fraud as early as possible in the customer journey. So, whenever a customer lands or opens an account, you have hundreds of data points to consider for your risk assessment. Whether you should allow your customer to be part of your customer base or whether you should implement some way of friction, you can delay specific verification steps until the customer becomes actually quite suspicious or when they hit some of the AML thresholds, given their activity, and this is also connected to detecting the monitoring activities which are, as I mentioned before, increasing.
It is increasingly challenging to detect and working with the regulatory bodies is an ever-evolving landscape, and the dynamics are also quite fluid. When I mentioned the wide range of data points that you can use to assess risk, the very first step is to look at your customer’s digital footprint.
So that’s what we have been providing to most of our customers at SEON to see whether the customer is using a brand new or disposable email address or is using any form of proxies such as VPNs as well as just the device itself can be used to see who is using the same browser or device. We can pinpoint whether the customer is using any form of emulation, virtual machines, or a suspicious browser profile.
A lot of activity is around automation-led attacks. So, fraudsters are increasingly investing in creating bots and scaling up their operation. Their aim and goal is to have the highest possible monetary value created by the least amount of effort and activity.
So that’s their goal in a short summary. Basically, when the customer is in and based on the initial assessment during onboarding, the way how a platform should work is, as I mentioned, if you can collect in your database and table or if you can also send these authentication-related points, such as login, details change, as well as if you have a digital wallet, you know, deposits, withdrawals.
Every time when you are able to assess risk is a good point to store user information and activity more in-depth. So then you can build the best possible models or let your social provider also tap into those data points and train the model, which is a vital part of machine learning. You can only make good rules if you are working the rule-based system as the data you have access to. We are capable of monitoring all these activities and then utilizing machine learning in order to provide these rule recommendations that can be supervised by a human.
So our customers can always overwrite and rule out some of the rules if they don’t like it. But we always have backup statistics given the confusion matrix results. So you can see how many good transactions you would block if you were to turn on any of these rules and as well as you can see how many of the actual fraudulent transactions you would block.
So that’s very important. Every time you create a rule or change some part of the decision-making logic, when you classify the users and the user activity into, you know, approving or denying those attempts and customers, then you have to make sure that you have in place, as well as the algorithm is actually working with the least amount of false positives.
And that’s where it can get really challenging, and the idea is really just to try to balance customer experience and friction. I think many of the new upcoming online leaders in this segment are trying to enable this so-called dynamic friction when you delay friction verification points, such as ID verification or phone or email-based verification, to when it’s ultimately necessary.
So the best is a friction-free or invisible type of risk assessment that’s also part of SEON’s core services. And then, based on this friction-free score or class that the system recommends, you can enable other types of verifications just for those customers who really seem suspicious, based on their behavior or attempts and as well as the complete profile.
And then, the point is to try to combine machine learning and human-made decisions. So, what we see from the market is that many of the fraud and risk and compliance teams are a bit worried about decisions made fully by automation and AI. So there is always a need for a human element in the process when someone actually reviews those transactions and also reclassifies them if necessary to enable the training model to make then better decisions based on the feedback loop.
Moving on to the next slide, I mentioned AI and whitebox machine learning. These are actually some of the fastest and most efficient methods of detecting fraudulent risky behavior; this can lead to reduced manual review times. If your decision-making algorithm is powered by AI and is continuously learning from decisions made by humans, then you can augment the best of the two worlds using AI and human-made decisions.
This can also provide you just better predictions on larger data sets. If you have the right labels, which then enable the best possible training data set, it can lead to a more efficient operation within your fraud risk and compliance team and a more cost-effective approach.
And then, when I mentioned whitebox machine learning, this is just a way to provide explainable human-readable rule suggestions. Many of the providers out there are actually providing blackbox type of decisions where you don’t really understand the specific science or the specific factors and how much they weigh into a specific decision.
When we designed our own platform, we always kept in mind that a human should be able to eventually make a decision over the rule, whatever the AI system would recommend. And this is why we believe that whitebox is such an important element of it.
I mentioned friction-free experience. This is siper important as user experience is one of the key selling points for many online businesses out there. They don’t want to increase churn, given all these friction points. You could do identification for every new customer, and you should if there is a regulatory requirement, but in many cases, that’s actually not the point. Identification on its own is actually not bulletproof because many fraudsters can buy stolen identities, stolen ID scans.
And then it’s quite expensive as well. Social providers for identification can charge up to one or even two US dollars for every check. And as well it’s a friction in the onboarding process and this can lead to churn. So that’s why friction-free, invisible risk assessment comes into play and actually lets you only do identification when it’s ultimately a must-have.
I mentioned that finetuning models is the basis and the foundation of making the best possible decisions. Unless you have the right labels and training data sets, you will have a very rigid, rule-based engine. What we try to enable our customers is that every cluster and group of fraudsters can be the basis of these rule recommendations.
And the system can actually provide you insights into what new rules you should turn on, what rules you should change, and what parameters are currently increasing your false positive ratios. When you have all these elements in place, then your team can actually focus on the most effective type of activities.
For example, making sure that the customers you’re labeling are actually really the right ones and are really fraudsters. It is also important to connect the systems and make sure that decisions are made by your vendor or different kind of proactive heads up you hear from, your card issuers and card networks are actually reflecting on your model.
We have created a platform that actually retrains its model on a continuous basis, which actually flexes on decision accuracy, which is quite high. So if you would retrain your model only on a daily basis, then all those transactions that fall into the last 24 hours before retraining are actually only the ones you would capture.
If there is anything that comes after and before the next training period, then you lose out on the efficiency, and you lose out on those new insights and patterns that you could actually leverage to make sure that you have the most accurate real-time algorithm in place. When we look at the future of fraud prevention for payments, we always suggest to our clients that they should layer multiple comprehensive solutions.
They should consider the whole digital footprint, which includes the email, phone, IP and device, but of course, if they actually use identification, use the data points that can come from the vendor or your own solution and can lead to the widest range of data points.
As I mentioned in the very beginning, you can only make as good decisions as the data you have access to. So it’s super important to make sure you capture every single behavior point, and as well as every authentication point would be a step where you assess risk and factor in all these wide-ranging data points.
Our solution can provide thousands of these data points, but it’s an internet platform so we can provide you the best possible decisions. We have seen that experienced fraudsters are also operating like businesses. They try to make as much money and as much monetary gain as possible in the shortest amount of time.
We have seen an increased number of bot attacks, which are fueled by the new upcoming ways of automation. They are using headless browsers or sending scripts to actually automate the actions in a web application or in a normal mobile application. And then it’s very hard to detect those types of attempts unless you have some type of behavior analysis.
And then, in order to prevent those types of attacks, you also have to invest in automation and different tools that can detect these specific patterns, but as well as the processes are easily adopting these new types of tools, including AI, like GPT-based large language models. So, they can be used to scale up their operation to the infinite.
This is where the whole subject gets really challenging. So, machine learning can be the solution to fight machine learning, such as, you know, part of AI-based solutions. And I believe that the best tools out there are are actually starting from the very early access point to your platform.
So, onboarding is not just about monitoring transactions with existing customers. That’s also super important for AML and detecting account takeover attacks, but as well as, you know, the first step when the customers are filling out an onboarding form, letting the online business know about their email address and phone number.
This is a must-have step during the customer journey. And you have a chance at this point to actually collect all these wide-ranging data points that later can be useful to detect more fraudulent attempts on your own platform.
Essentially, if I boil down the whole picture into one single statement, I would say that as an online business, the most important role is that you actually have the highest amount of true positives and the lowest amount of false positives.
So, in order to do that, without having the right data set in place, having the right labels, and training your models to make the best possible decisions, it will probably be an uphill battle. If you are just considering onboarding or ongoing monitoring, then you are missing specific pieces of the whole customer journey.
So you have to look at the whole picture. You have to look at every attempt at every step of the customer. And then, when we work with large businesses like Revolut, Wise and Nubank, you see that they have their own data science teams. So they have access to this data. We try to let them leverage our data points and then use our model as well. This way, we can co-host the model with those teams. And this can lead us to having an ultimate silver bullet type of solution when they leverage additional data points and leverage their own data. Still, the decision is actually based on the combination of the two algorithms they have in place and also what we provide to them as suggestions.
The question is whether to buy or build. I believe that when you have access to extra data points, which are usually very hard to get, and as an online business, it might not be your core objective to actually collect data about an email or phone or device. It makes sense to use some of the point solutions, but then it can lead to kind of like a horrible effect when you have to build a middleware and use multiple vendors.
There’s an increasing need for all-in vendors because most of the online businesses out there are trying to consolidate their KYC stack. What you try to enable at SEON is to tick all the boxes of the needs of the risk and compliance team by building a platform that can serve them as an AI-driven solution and also as a system of record. So they don’t have to turn to multiple vendors or switch tabs when they’re operating as normal. So that’s all cool. And I hope this, gave some of the viewers a better picture of what should be the balance of the customer journey and how you can make the best possible decisions.
For the next step, I would like to open up for questions here.
Reece: Perfect. Thank you so much, Tamas. I mean, all the avenues discussed there were brilliant. Just starting with questions; if you have any questions, please leave them in the panel below. Again, we’ll forward them over to the team at SEON, and they’ll get back in touch with you, I’m sure of it.
I certainly wanted to start with the most common types of fraud SEON users experience on a daily and encounter. So we touched on that wide range and how machine learning is really integrating new techniques for fraudsters to kind of target people. What are the most common ones you’ve seen for SEON users?
Tamas: Yeah, I would say that every specific fraudulent attack is created by using stolen identities. This is also called identity theft. So even when we talk about payment fraud, where the real symptom is a chargeback, then usually it’s done by stolen credit card numbers, which is also a form of identity theft. The most common types of fraud are either stolen or synthetic identities are being used to make unauthorized transactions or open fraudulent accounts.
And I just see more ways are being utilized by fraudsters to collect the stolen identity pieces. Let’s say, with fake websites, fake e-commerce sites; they can act like a real e-commerce retailer where customers will think that there’s a great deal out there. They enter their credit card information, and it’s already stolen at that point, so making sure that the site is genuine and you have good reviews somewhere on the internet is super important.
I also saw cases where fake job postings were used by fraudsters to collect real IDs and passport scans of customers. And then these scans and passports were used to open up accounts. I think everyone who is online should be alert at all times to make sure that they don’t fall victim to any of these attempts.
Reece: Definitely. I spoke to Alex Wood, and he touched on what you mentioned today: how AI and machine learning can create a forward strategy from start to finish and doing this simultaneously thousands of times to target people. From this, the question is, what types of fraud are you seeing becoming more prevalent due to AI?
Tamas: Yeah, of course. So, we have seen other cases of specific AI-powered attacks. This can be, for example, the so-called CFO fraud where a deep fake can be used to seem like, you know, as a company’s CFO when they ask to urgently wire out funds to a so-called drop or mule account and as well as, you know, in the romance fraud type of attempts, which are also a type of social engineering attacks. The conversation can be automated by large language models. These can help fraudsters to scale up their operations to levels we have never seen before.
So imagine if you think that you were talking to a human on a dating app, that it can really just be a GPT-based script where you actually are talking to an AI, not a real person. This way, fraudsters can have thousands of ongoing parallel conversations where, in the end, they would try to push their victims to wire out funds or, you know, click on the link and enter some personal details. So, just the way how these phishing and social engineering attempts are run the same way with GPT-based AI models, the possibilities are infinite for fraudsters. So that’s why it’s super important to fight back with AI-based systems that can real-time predict the risk levels from those attempts.
So even if you are a fraud or risk analyst working for a business that does not even have a chargeback problem, you might have a problem with fake accounts being used to actually leverage your customer base to run scams, extort money, etc. Fraudsters are not only targeting e-commerce sites or digital banks but are targeting every possible place where there are social interactions because this is where you can actually utilize human-to-human-like conversations, but also, if you run an AI-based campaign, then you’re actually making some attempts to try to have as a wide cast as possible.
Reece: That was a great explanation of things, especially around romance scams and not just the loss of money but the impact of the mental health side of things and how that draws into the business as well that they’re working for, and so that was brilliant. And then, just a roundup final question and just kind of wanting to know a bit more about digital profiling and what that is.
Tamas: Sure. So, digital profiling for us is to collect all the publicly accessible data points based on your customer’s digital footprint, real-time have all this data in place, and basically, when I mentioned the data points customers are entering on onboarding forms, such as email address or phone number, or even address, as well as their device and IP, you can have thousands of additional data points. You can see whether your customer is using a new email address, trying to hide behind a proxy, or using a malicious device.
And when you have this data at your fingertip, then you can analyze the data and then increase your decision-making algorithm. You can provide a more friction-free way of authentication. I think it’s a crucial tool for fraud prevention. It can help to identify inconsistencies or anomalies in your user activities, which can then lead to an indication of fraudulent behavior.
I think it’s a new way of enabling this so-called dynamic friction-based customer journey where you can actually delay that friction verification method to the latest point possible.
And also, you can save a lot of money by not doing verification for every new customer if the risk level is really low.
Reece: Amazing. And that was a great overview of three questions there. If you do think of anything after the recording, please do drop Tamas a message on LinkedIn as well. I’m sure he’ll be happy to connect and speak with everyone who’s attended, but that kind of rounds up the Q&A segment for this. So, if you don’t mind forwarding it to Tamas, thank you.
That brings us to our next Wired-In webinar, which is going to look into the state of compliance in 2024. We did a massive research report interviewing over 120 compliance professionals. And we’re going to look to share these insights with our chief product officer and head of customer success here at NorthRow. So keep an eye out for these emails. We’re going to be looking to host that next month. And then one more forward, please, Tamas.
Fantastic. So that’s just a big thank you to Tamas and everyone at SEON who’s helped make this happen. I hope everyone found that really insightful. Please do check out SEON for your fraud needs. That kind of brings everything to a close, and a big thank you again. Thank you everyone for joining.
Speak with a fraud fighter.
Click here
SEON Resources
Sign up for our newsletter
The top stories of the month delivered straight to your inbox